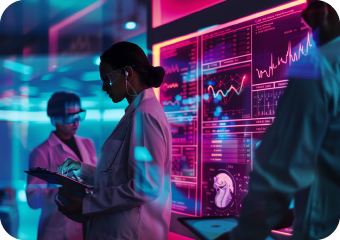
The mortgage lending industry has leveraged AI historically to help drive efficiency and customer experience. However, reliance on supervised learning and limitations on creativity limits these benefits from traditional AI. Bloomberg Intelligence findings state that Generative AI (Gen AI) will help expand the mortgage market at a CAGR of 42%, growing from $40 billion in 2022 to $1.3 trillion over the next ten years. This article highlights the potential for leveraging Gen AI to transform mortgage lending business operations.
The mortgage industry is gradually beginning to leverage Gen AI, and the rate of adoption among mortgage lenders could use some additional traction, as evidenced in the findings from the Arizent survey. It is, however, expected that the increase in the number of LLM (Large Language Model) providers and the capabilities offered by these models will help drive increased adoption in the future. While there are encouraging signs of some lenders beginning to embrace this cautiously, it’s critical for those not yet considering Gen AI as part of their technology strategy to recognize that delaying adoption could prove detrimental.
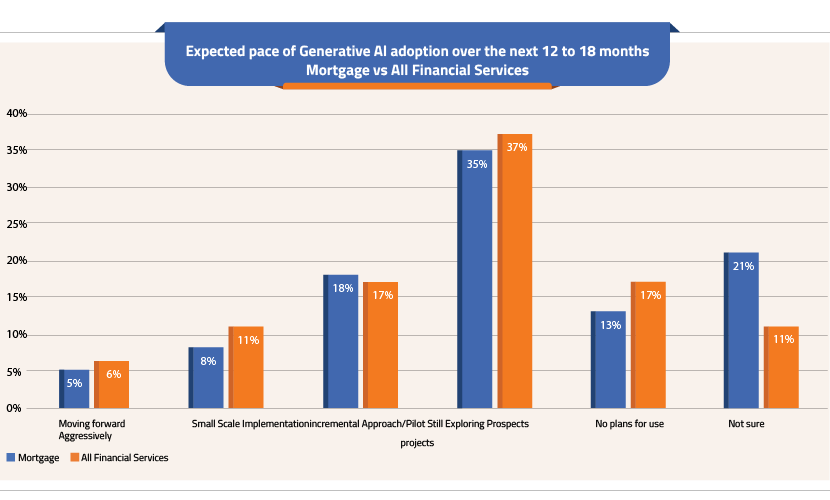
A. Streamlining Correspondent Lending with Gen AI
Most large US lenders have correspondent lending relationships with smaller banks that sell their loans to maintain cash flow. The process typically involves assessing risk before purchase by reviewing loan packages that are typically 150-250 pages. Reviews are comprehensive and cover insurance, collateral, borrower/loan, and compliance, with users spending about 30 minutes on each review. On average, loans undergo 5-7 reviews, totaling ~4 hours/loan. Pre-processing involves manual data preparation by operations teams or leveraging OCR (Optical Character Recognition) from third-party vendors. While the review process is standard, the loan characteristics may require detailed review. Gen AI provides immense potential to accelerate the review process and streamline loan purchases.
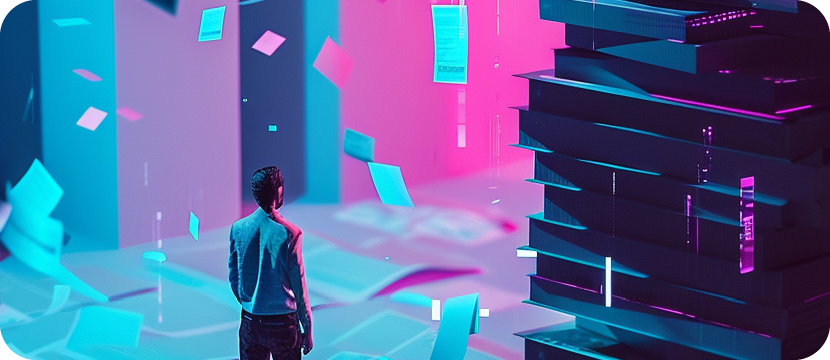
-
Transforming OCR with Gen AI: Accuracy Redefined for Document Extraction:
Traditional OCR solutions by leading mortgage technology companies rely on pattern recognition algorithms with rules to convert extracted text into machine-readable form. The poor image quality of scanned documents and the varying formats for non-standard documents like income statements, credit reports, and insurance records pose challenges in processing. While traditional OCR performs well with standard documents, it has challenges with non-standard ones, dropping the accuracy to 70-80% despite vendor claims of >90%. The emergence of Gen AI, particularly with newer LLMs, has revolutionized OCR by enabling learning and improvement. While the jury is still out, OCR tools from leading LLM providers demonstrate promising accuracy upwards of 99%. This provides Correspondent lenders a significant opportunity to leverage Gen AI-powered OCR for enhanced data extraction and automation.
-
Empowering Lenders with AI-Driven Insights for Informed Decision-Making:
The correspondent lending process is crucial for ensuring a quality mortgage loan portfolio. Loan data made available through extraction or operational processes undergoes comprehensive reviews to meet the bank’s underlying criteria. These reviews typically include a series of checks to cover insurance coverage, qualified mortgage, compliance, collateral/property, borrower qualification, and appraisal. Each review is handled by individual departments, with users logging into the application to assess data points. Generating a suspense notice to the correspondent lender determines whether loans are approved or require additional conditions to be met. Gen AI has immense potential here to help generate suspense notices, which contain the total sum of all conditions added by each reviewer.
B. Transforming Mortgage Customer Experience with Gen AI
The ability to provide customer support via chatbots is critical in retail mortgage to allow customers access to periodic information updates. Unlike opening a checking or savings account, mortgage cycles typically take 30-60 days to close. Lenders need to build customer confidence during this time and avoid lapses in communication that could damage relationships and hinder cross-selling opportunities. Traditional chatbots rely on NLP (Neuro-Linguistic Programming) and rules based on extracted keywords, often leading to unsatisfactory responses. Gen AI-powered chatbots, however, use LLMs trained on vast amounts of data and customized to the lender’s environment, providing a more human-like experience.
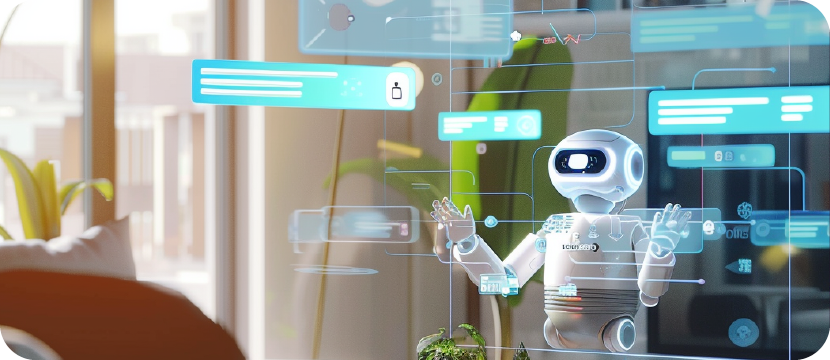
C. Accelerating Property Appraisals with Gen AI
Mortgages typically can take 30-60 days to close, largely due to the time-consuming appraisal process, which can take up to 2 weeks. The appraisal reports can vary in their nature and are a function of the type of property type and resulting inspection. While some automated solutions exist, appraisals require a thorough assessment of multiple factors, including property types, location, flood zones, neighboring property values, and legal guidelines. Gen AI has immense potential to generate accurate valuations by processing vast amounts of data from Large Language Models, including past sales, market trends, and neighborhood characteristics. Combining these insights and using vision algorithms to identify anomalies in property images and area data provides a compelling way to have Gen AI produce accelerated appraisal reports, facilitating faster mortgage closings.
Elevating Mortgage Correspondent Lending Reviews with Gen AI: Key components
The Mortgage Correspondent Lending Review Use Case is heavily influenced by the review parameters defined at the lending bank. While Prompt Engineering helps with basic understanding, fine-tuning a foundation model is recommended for this unique use case. Here’s the proposed solution overview:
- Selecting the Optimal Foundation: Choose a model that aligns with the enterprise architecture and expectations to avoid building something from scratch, which would be expensive and time consuming. The idea is to have a base model already trained on a vast corpus of text, retaining a broader context comprehension.
- Crafting the Perfect Dataset: Create a dataset specific to the use case, focusing on factors reviewers could flag as conditions for correspondent lenders to act upon. Convert standard parameters and actions, historical out-of-box conditions, and key loan review parameters into Prompt-Completion pairs. This is currently a manual process, though tools exist whose efficacy is not yet validated.
- Fine-Tuning Models for Peak Performance: Train the foundational model on the prepared dataset to recognize patterns and relationships within the data. Validate the fine-tuned model’s success by its ability to generalize without overfitting; this typically occurs when an algorithm fits too closely to its training data, resulting in a model that can’t make accurate predictions or conclusions. Through iterative testing and fine-tuning, the model will learn to review loans, determine approval status, and generate suspense notices.
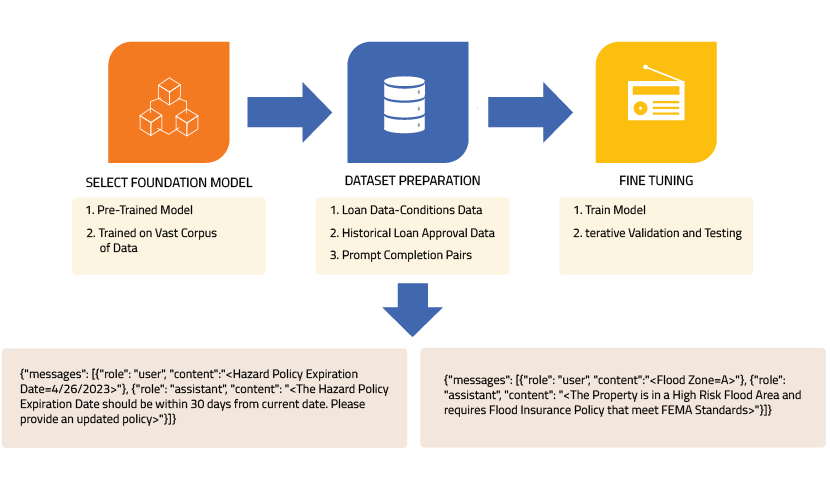
Key Considerations in Adopting Gen AI
As is the case with any new technology, the adoption of Gen AI raises concerns about privacy, security, compliance, and IP while its scope and options continue to expand rapidly. Banks and lenders must consider the following when embracing the Generative AI space: to leverage the technology effectively and put it to good use.
- Open-Source Vs. Proprietary: As with most IT solutions, selecting open-source players like Open AI and Meta AI versus proprietary/closed-source players like Google and Microsoft will play out for dominance. The selection of the right Gen AI tool/platform should be based on a comprehensive analysis of factors like pricing, integration capabilities, performance, ease of implementation, time to market, and security, tailored to the business’s specific requirements and long-term objectives.
- Data Availability: The success of any AI initiative depends on the quality of the data (and the models). While current models like GPT-4 are built on vast amounts of data, they don’t address every industry-specific question. Fine-tuning the model to specific use cases and requirements requires enough data and domain knowledge.
- Security: Security is paramount in Gen AI implementation, particularly in the mortgage industry, which deals with sensitive personal data. Lenders should thoroughly assess providers’ security frameworks and build guardrails on data handling. Tokenization can help convert human language into machine-readable language, protecting PII (Personally Identifiable Information).
- Enterprise Landscape: Adopting Gen AI should align with enterprise goals. Continuing with existing cloud or analytics providers can ensure operational continuity and ease of integration. The role of domain SMEs and operational resources is key in validating the success of the fine-tuned model, making it more than just a technology implementation.
Loved what you read?
Get practical thought leadership articles on AI and Automation delivered to your inbox
Loved what you read?
Get practical thought leadership articles on AI and Automation delivered to your inbox
The Gen AI- Led Future of Mortgage
The mortgage industry has started embracing Gen AI, primarily through a pilot of chatbot use cases. However, there are broader applications where Gen AI could be a game-changer and disruptor, as outlined in this paper. Additional areas within the mortgage industry, such as rate sheet generation, reporting, application tracking, and cross-selling among other things, also have the potential to leverage Gen AI and derive the benefits of increased efficiencies and cost savings as well. The winners will be those who take bold and measured steps in their technological adoption to lead the way with Gen AI.
Disclaimer Any opinions, findings, and conclusions or recommendations expressed in this material are those of the author(s) and do not necessarily reflect the views of the respective institutions or funding agencies.
- https://www.bloomberg.com/company/press/generative-ai-to-become-a-1-3-trillion-market-by-2032-research-finds/
- https://www.nationalmortgagenews.com/list/mortgage-industry-expresses-concerns-and-hopes-over-ai-use
- https://research.aimultiple.com/ocr-accuracy/
- https://arxiv.org/abs/2310.16809
- https://themortgagereports.com/19487/how-long-does-it-take-to-close-a-mortgage-gina-pogol
- https://www.ibm.com/topics/overfitting
- https://medium.com/@attriai/choosing-your-path-in-generative-ai-open-source-or-proprietary-40b6edb3ac57
- https://www.protecto.ai/blog/counter-gen-ai-risks-with-data-tokenization