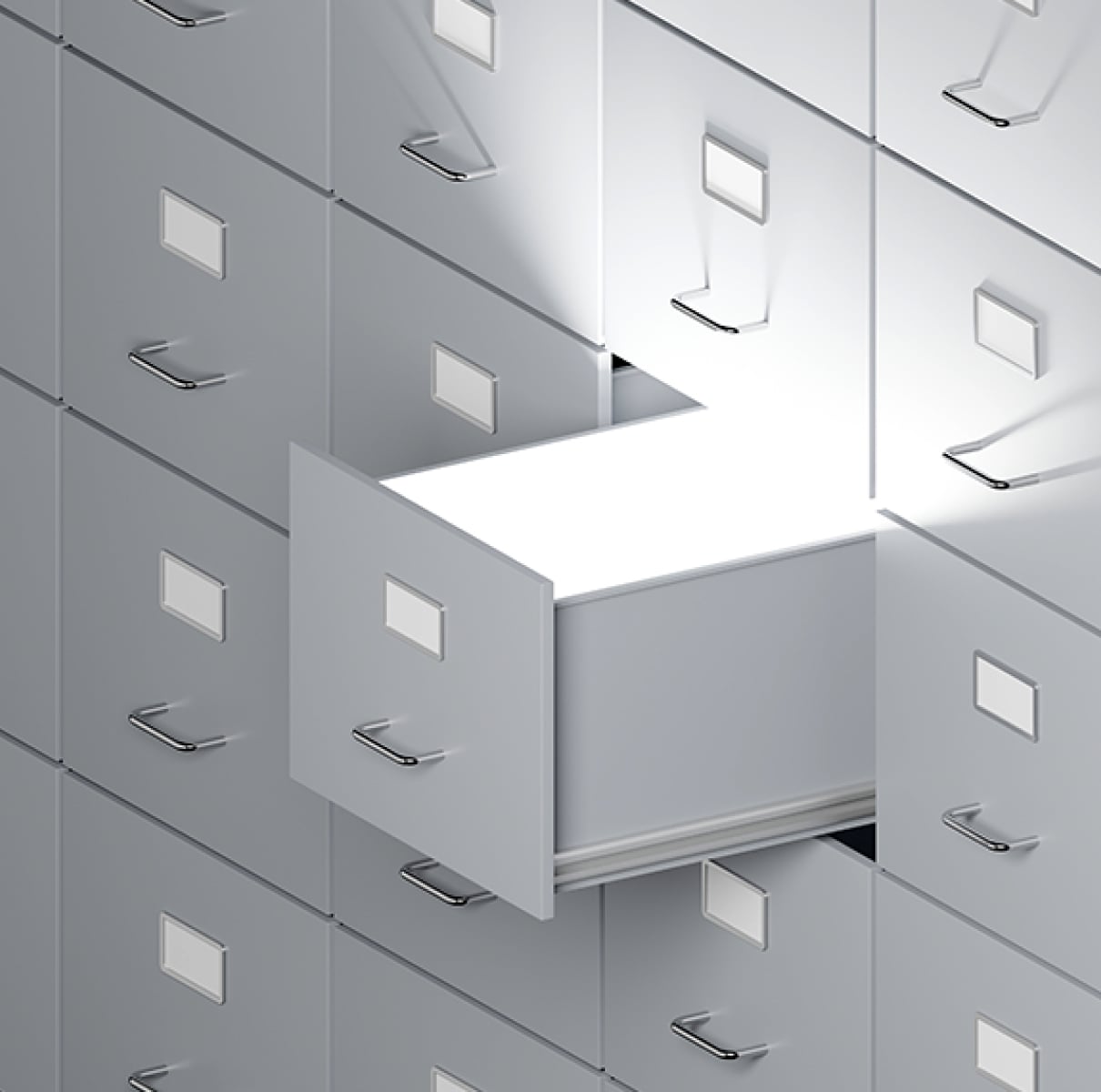
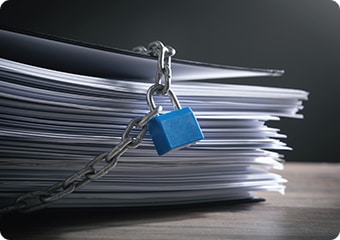
Do you have a substantial amount of unstructured information sitting within documents on your intranet? If so, you are not alone. Although unstructured data is a major contributor to information overload, it cannot be ignored. There is a need to realize that the story is not about document structure and kind; but about the unstructured data and insights trapped in these document types. Read on to know how insights from these documents can help deliver greater value.
Media and telecom (M&T) leaders are waking up to the value of unused unstructured data in their organizations. Moving beyond the traditional use cases of document digitization, such as invoice processing, order form processing, etc., they have started looking at unstructured documents in a new light. They want to uncover new insights from millions of these documents to find answers to pressing business issues such as:
- How do we reduce costs on legacy network operations?
- How do we optimize power tariffs or tower lease rentals?
- What is the revenue assurance for enterprise billing is given decades-old contracts with 100s of amendments?
- How do we respond efficiently to subpoenas?
- How do we ensure contract compliance with and from our partners?
Unfortunately, unstructured data’s unique properties make extracting relevant, accurate, and timely insights challenging. Recently, we had a conversation with a telecom company that wanted to understand1 the threat of eviction based on their tower lease agreements. They had three million documents of 32 document types. It was almost impossible for them to get any usable insights from the more commonly used OCR-based methods.
Traditional OCR-based approaches are suitable for text extraction but fail when presented with tables, charts, logos, etc. In addition, document volumes amplify the need for high accuracy and straight-through processing. Poor-quality legacy documents become even more challenging for traditional document digitization models to process.
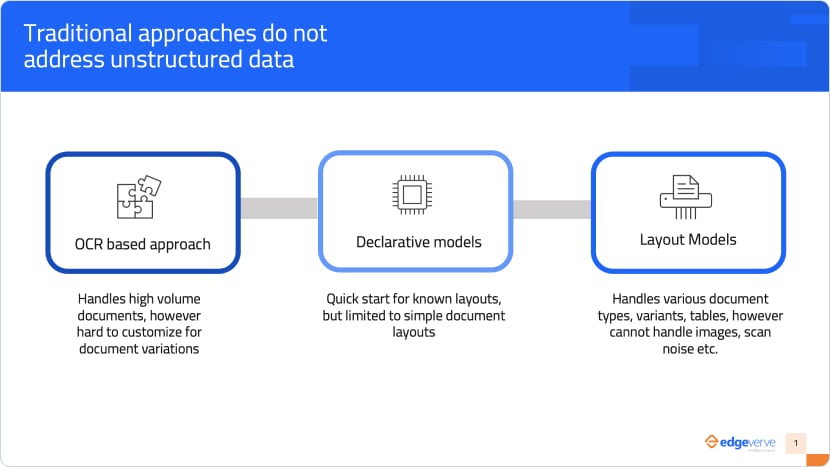
It is no longer enough for document processing solutions to identify areas on a form and extract individual fields – even when documents are natively digital. Taking a layout-based approach to determine field values isn’t a scalable option. It fails quickly in the face of unstructured data such as logos, images, multimedia files etc.
With unstructured data volumes growing 3x faster than structured data, these challenges will only escalate if enterprises look at new approaches.
To be business-relevant, data processing solutions need to extract the right sentiment and fields with the right context so that a downstream human, bot, or system can consume it. For example, a billing system needs to know the relevant pricing terms for a specific service to generate an accurate invoice. A bot will need to have a trigger to execute any action. And even a human will need the right information to take appropriate action. For instance, to plan a PR campaign, the public relations team for an entertainer will need to know the audience’s sentiment – what the news and media are saying, are the reviews positive or negative etc.
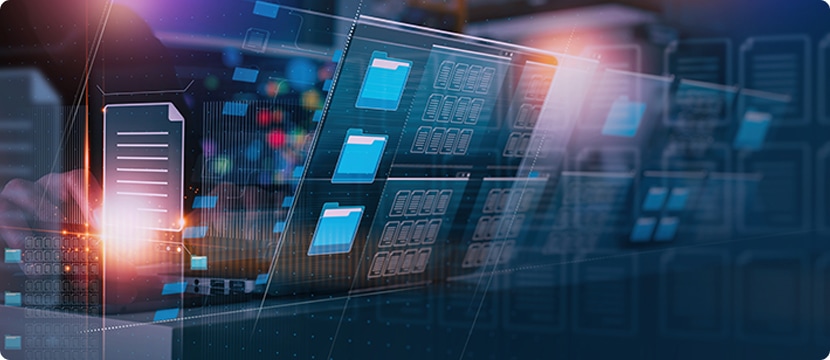
While there has been much interest in document digitization post-pandemic, we see much undue focus on document types. There is a need to realize that the story is not about document structure and kind; but about the unstructured data and insights trapped in these document types.
The right technique enables faster extraction of relevant data to the highest level of accuracy by optimizing for:
- Volume – number of documents processed for a use case
- Variety – variations of document structure, location of information, info hidden across documents, types of unstructured data like checkboxes, tables, lists, logos etc
- Velocity – how fast can relevant data be extracted – from real-time to batch
To get productivity benefits or impact revenue, unstructured data must be consumed to derive insights, drive action, or optimize automation. Unfortunately, most systems cannot use this extracted information in the native form; it usually needs some transformation. For instance, a system, human, or bot will seek insights on “what is my supplier risk based on existing contracts?” or “Why didn’t the client pay the billing invoice?” To answer these questions, the extracted data must be post-processed for downstream systems. This is where the ROI is.
The critical thing for scaling these solutions is to remember not to mix extraction and consumption – don’t apply rules for end consumption during extraction.
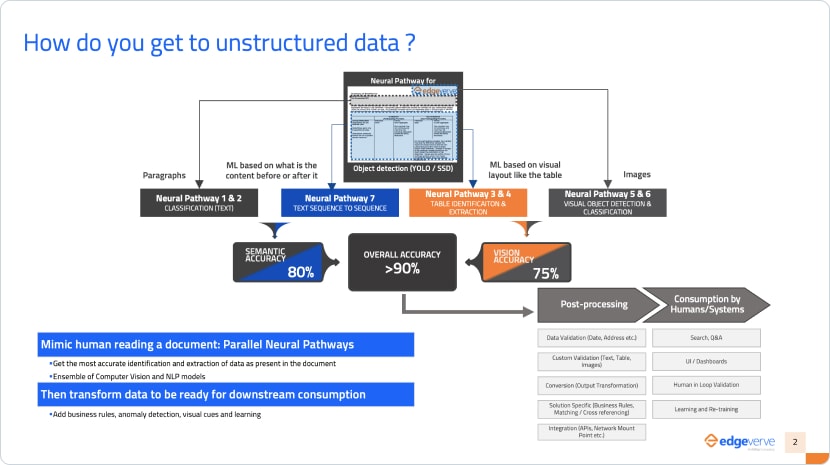
AI-based document processing should mimic human behaviour. Think about how you process unstructured data. Let’s say; for example, you look at a contract at work. You don’t immediately start reading it word by word. You look at the entire document structure, determine where the information is, gauge which parts of the contract are critical to you, and how different colours, imagery, logos etc., are used. You comprehend the document through multiple neural pathways. Extracting unstructured data with AI-based IDP is similar.
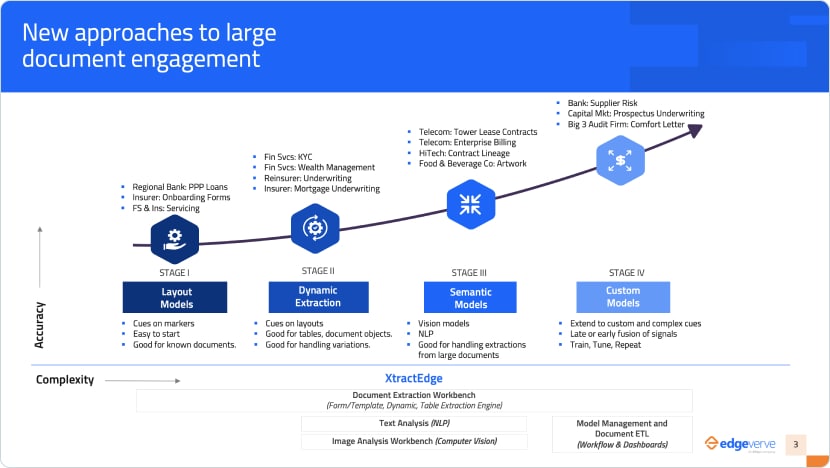
In AI-based IDP, an ensemble of models – computer vision models, natural language processing models, table extraction models, etc. – are simultaneously applied to the document. An ideal platform would:
- Tune the output of each of these models across different extractions to get the highest accuracy extraction of one element – a logo, a table, an image etc.
- Constantly validate everything it extracts to meet high accuracy expectations
- Post-process this data with domain, company, and use-case-specific rules and make it suitable for downstream consumption.
- Provide telemetry to improve performance and accuracy – at what level do you need more inputs, is the accuracy trending lower, or do you need to train the human or the machine?
- Be domain-agnostic, highly trainable, and flexible enough to mould outcomes for any use case.
- Should be able to add human-in-the-loop to manage exceptions easily and, over time, improve the system’s performance.
The right platform will solve the unstructured data problem end-to-end – from different ingestion techniques, sources, and how businesses consume it.
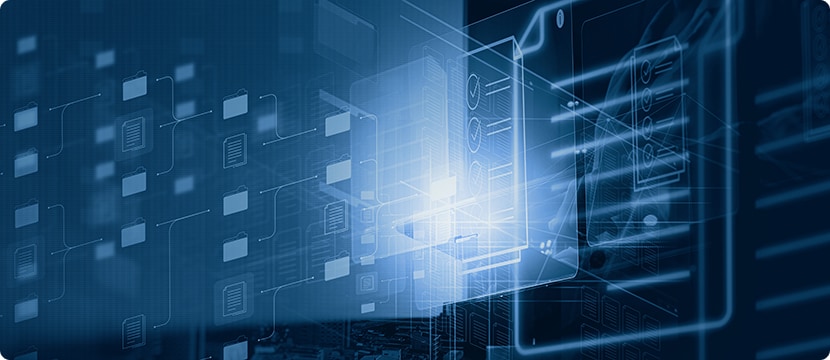
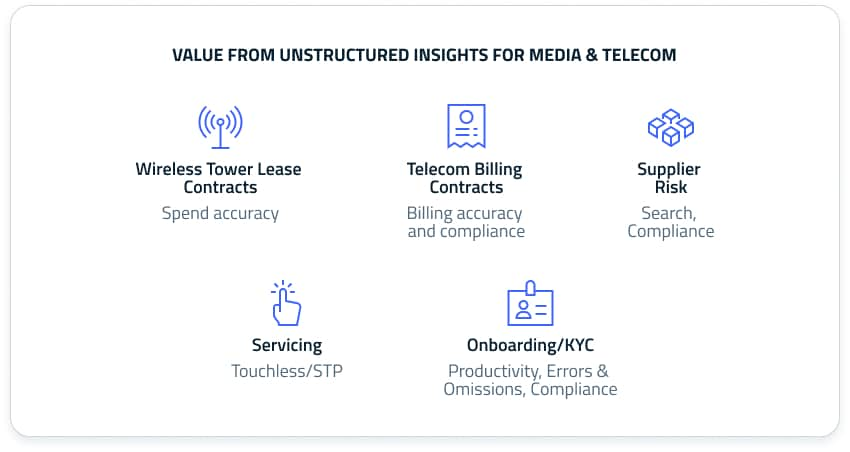
Relying on human interventions to unlock insights from unstructured documents creates productivity issues, revenue leakage, compliance concerns, and impacts speed to revenue. Automated extraction of consumable information can go a long way in solving these problems.
Loved what you read?
Get practical thought leadership articles on AI and Automation delivered to your inbox
Loved what you read?
Get practical thought leadership articles on AI and Automation delivered to your inbox
Document digitization is the stepping-stone to scale automation across your enterprise. In a world that is rapidly moving to end-to-end automation, the right solution for document processing is going to be crucial for holistic digital transformation. The need of the hour is a solution that can process millions of business documents to deliver the desired outcomes quickly, efficiently, and economically.
To really unlock business value with IDP, look beyond traditional use cases and dig deeper to uncover use cases embedded in business operations that can improve revenues or costs. And then look for a technology that can support all these various use cases.
Disclaimer Any opinions, findings, and conclusions or recommendations expressed in this material are those of the author(s) and do not necessarily reflect the views of the respective institutions or funding agencies