COVID-19 has wrought unprecedented disruption for global supply chains, with 75% of companies reporting some form of disruption, including longer lead times and delays in receiving orders1. Images of empty shelves, deserted airports, etc. splashed around social media bring home the grim reality. According to data from supply chain management platform Tradeshift, trade has flatlined in regions under lockdown, and the effects will continue to linger in the coming months2.
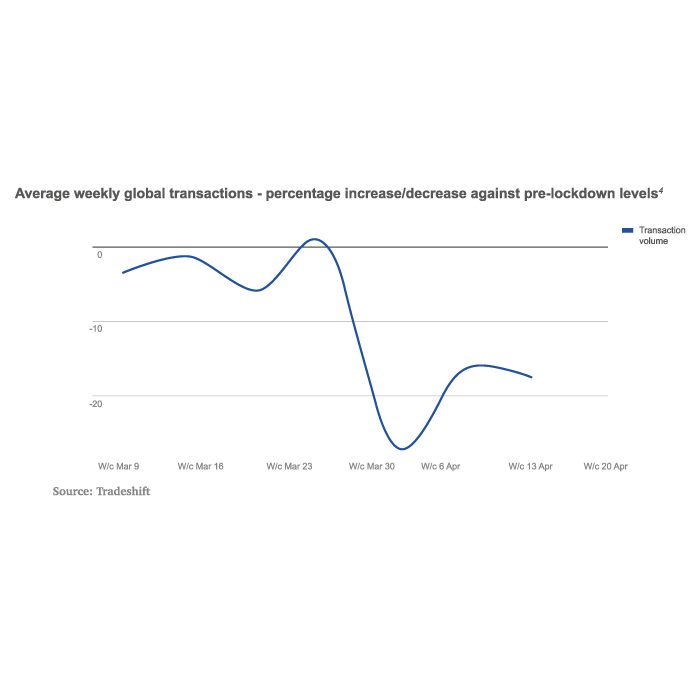
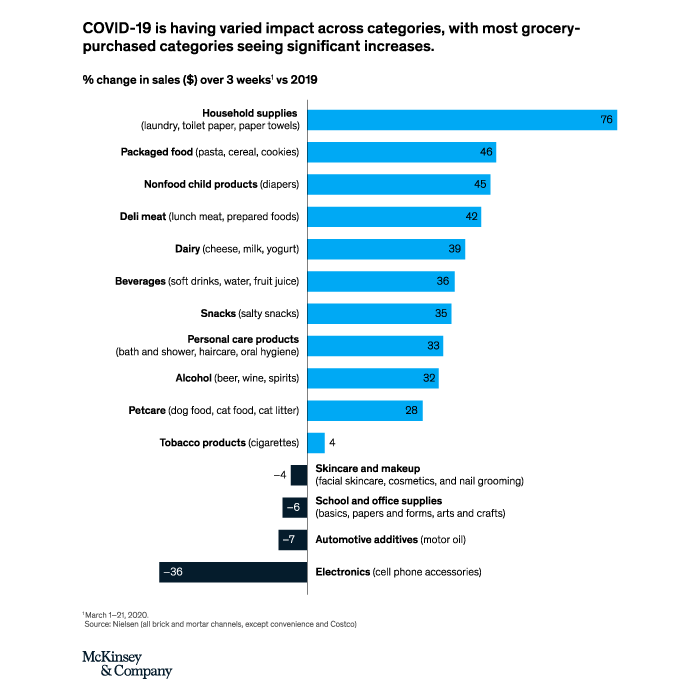
Steps to be taken by retailers and CPG manufacturers to combat the crisis
EVALUATE | ASSESS | RECALIBRATE | RESPOND |
---|---|---|---|
Evaluate the extent to which changes in consumer behavior will impact their categories, channels, and brands during and beyond this crisis. | Assess what actions they can take now to calibrate their demand forecasting models quickly. Adjust for the forecast errors that are happening and will continue to occur in the models’ forecasting horizons. | Recalibrate supply and demand levers to improve business performance while also preparing for the new consumer behaviors which will be the ‘new normal,’ post-crisis. | Respond to this highly anomalous behaviour of COVID-19, due to which their traditional forecasting models are over-correcting their forecasts. |
A quick guide to forecasting and planning
Forecasting and planning are imperative, especially for retail and CPG business. These are the methods that help create strategies and tactics to achieve business goals like sales targets and inventory sell- throughs.
There are numerous benefits of forecasting and planning; for instance, an analysis of purchase pattern history and projections of future customer demand can help retailers manage channel store merchandise inventory. Planned daily optimizations for price, promotion, and markdown, among others, ensures optimal profitability irrespective of the season. Forecasting and planning also help optimize shelf space, product fulfillment, and manpower. Accurate forecast of demand – by the hour, day, location, promotion, and price change, can help make important decisions pertaining to inventory, staffing needs, call centers, and fleet crew.
Forecasting : Mark Twain said, “History doesn’t repeat itself, but it often rhymes.” Forecasting looks at historic behavior with an assumption that the future is a repetition of the past. Controllable historical events (like promotions) and uncontrollable historic events (like the SARS outbreak, or the present COVID19 pandemic) can be considered during modeling. The insights from forecasting are invaluable for companies in demand planning.
According to one of the world’s leading experts in forecasting sciences, Dr. Spyros Makridakis’ forecasting techniques fall into two major categories – quantitative (focused in this article) and qualitative.
Quantitative Forecasting Methods
- Time-Series: Predicting the continuation of historical patterns such as the growth in sales or gross national product (time-series or temporal data)
- Causal / Econometric Modeling: Understanding how explanatory variables such as prices and advertising affect sales (cross-sectional data)
- Combination of both approaches: Models that involve both time series and causal modeling approaches, e.g., dynamic regression models, state-space models, etc.
Best suited to the present times, time-series forecasting can be type-casted as a supervised learning problem. It can be used with machine learning (ML) and deep learning (DL) based methods such as Regression, Neural Networks (RNN/CNN), Support Vector Machines, Random Forests, XGBoost, etc.
However, time series forecasting using ML/DL have their own set of unique quirks – such as the need for retraining the ML/DL model every time you want to generate a new prediction and the need to do away with train/test splits7. This requires data-scientists & ML engineers to rethink their approaches to building, evaluating, and re-calibrating models.
Not only point-forecasts, but the uncertainty of the forecast (also known as “prediction intervals”) are equally as important, or even more so in some cases, than the forecast or the point-estimate itself. In demand forecasting and inventory applications, the uncertainty of a forecast is a critical input for the applications/software that consumes it.
Typically, there is an iterative process to be followed for each step of the forecasting cycle: obtaining the latest data, generating updated forecasts, fixing problem forecasts, conferring with other internal or external parties about the forecasts, adding ad-hoc adjustments based on business knowledge, obtaining executive review and change approval, and publishing the forecasts. These forecasts are then typically used for production planning purposes, supporting marketing plans (promotion planning), resource planning, and financial planning and reporting.
- Planning : While forecasting focusses on what will happen, planning deals with what should happen, under the constraints of available resources in making it happen. Planning techniques involve specific algorithms such as goal-seeking, what-if analysis, and optimization and operations research methods (See box for details).
Planning Techniques
- Goal-Seeking: This technique is valuable for defining fact-based plans. It requires advanced forecasting models that consist of both “inputs” (like historical business drivers, macroeconomic/weather data, and calendar events) and an “output” (which is the variable to be forecasted). Goal-seeking algorithmically varies the future values of the “inputs” in order to determine the values that achieve a specific goal (profit, revenue, or cost goal) based on the forecast-metrics. This helps in understanding how inputs need to be modified in order to achieve a certain goal.
- Scenario and Sensitivity Modeling (What-If analysis): The aim is to figure out the impact of changes in the inputs on the output. In scenario analysis, the user modifies the future values of the inputs to specific values and then evaluates the effect on the forecasts.
- Optimization and Operations Research Methods: The objective is to arrive at the most optimal scenario from all possible combinations of inputs. Optimization algorithmically varies the future values of the inputs to find the optimum of an objective function (profit, revenue, or cost function) based on the forecast model.
Planning helps companies answer questions like: What is the optimal mix of the sales price and advertising spend that achieves a pre-specified sales target or inventory sell-through? What happens to predicted sales numbers once the organization increases the sales price and decreases advertising spend (as in the scenario of a crisis like COVID-19)? What is the optimal sales price and advertising expenditure trade-off that maximizes profit margins and inventory sell- throughs?
Answering these questions has become very important for businesses struggling to survive this pandemic. However, using legacy forecasting and planning methods is not going to work in these unprecedented times.
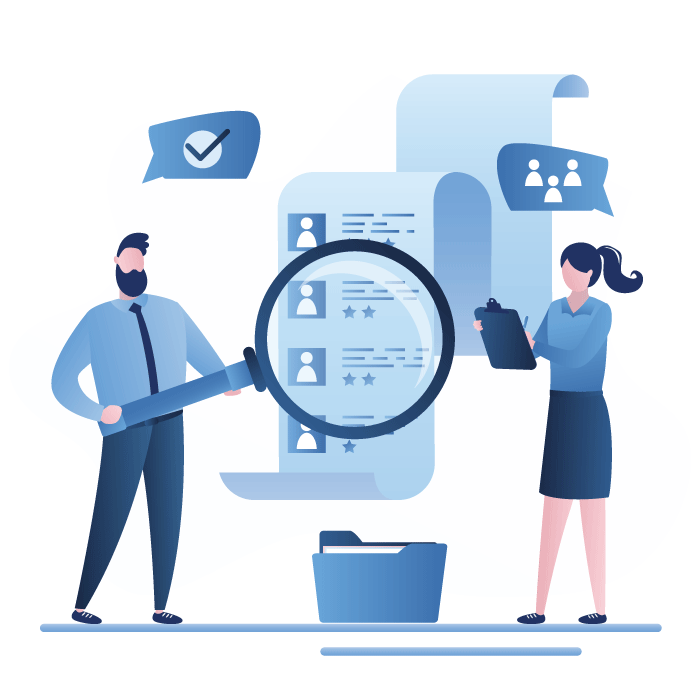
The COVID-19 crisis has tested the resilience of every business, and those with strong foundations in data-driven decision making are adapting to the change and recovering faster with strategies suited to the situation. Even when the crisis is past, data discipline will hold organizations in good stead – no matter what the circumstance.
References:
- https://www.weforum.org/agenda/2020/05/this-iswhat-global-supply-chains-will-look-like-after-covid-19/
- Image: https://www.weforum.org/agenda/2020/05/this-iswhat-global-supply-chains-will-look-like-after-covid-19/
- Image: https://www.weforum.org/agenda/2020/05/this-iswhat-global-supply-chains-will-look-like-after-covid-19/
- Image: https://www.weforum.org/agenda/2020/05/this-is- what-global-supply-chains-will-look-like-after-covid-19/
- https://www.mckinsey.com/industries/consumerpackaged-goods/our-insights/rapidly-forecasting-demandand-adapting-commercial-plans-in-a-pandemic
- https://www.edgeverve.com/the-edge-quarterly/wheresight-meets-might/