AI In Mobile
The Possibilities Ahead
Heinz Riehl Chair Professor of Business, Professor of
Technology, Operations, and Statistics, NYU Stern
School, Professor of Marketing, NYU Stern School &
Director of Masters in Business Analytics, NYU Stern.
AI In Mobile
Technology, Operations, and Statistics, NYU Stern
School, Professor of Marketing, NYU Stern School &
Director of Masters in Business Analytics, NYU Stern.
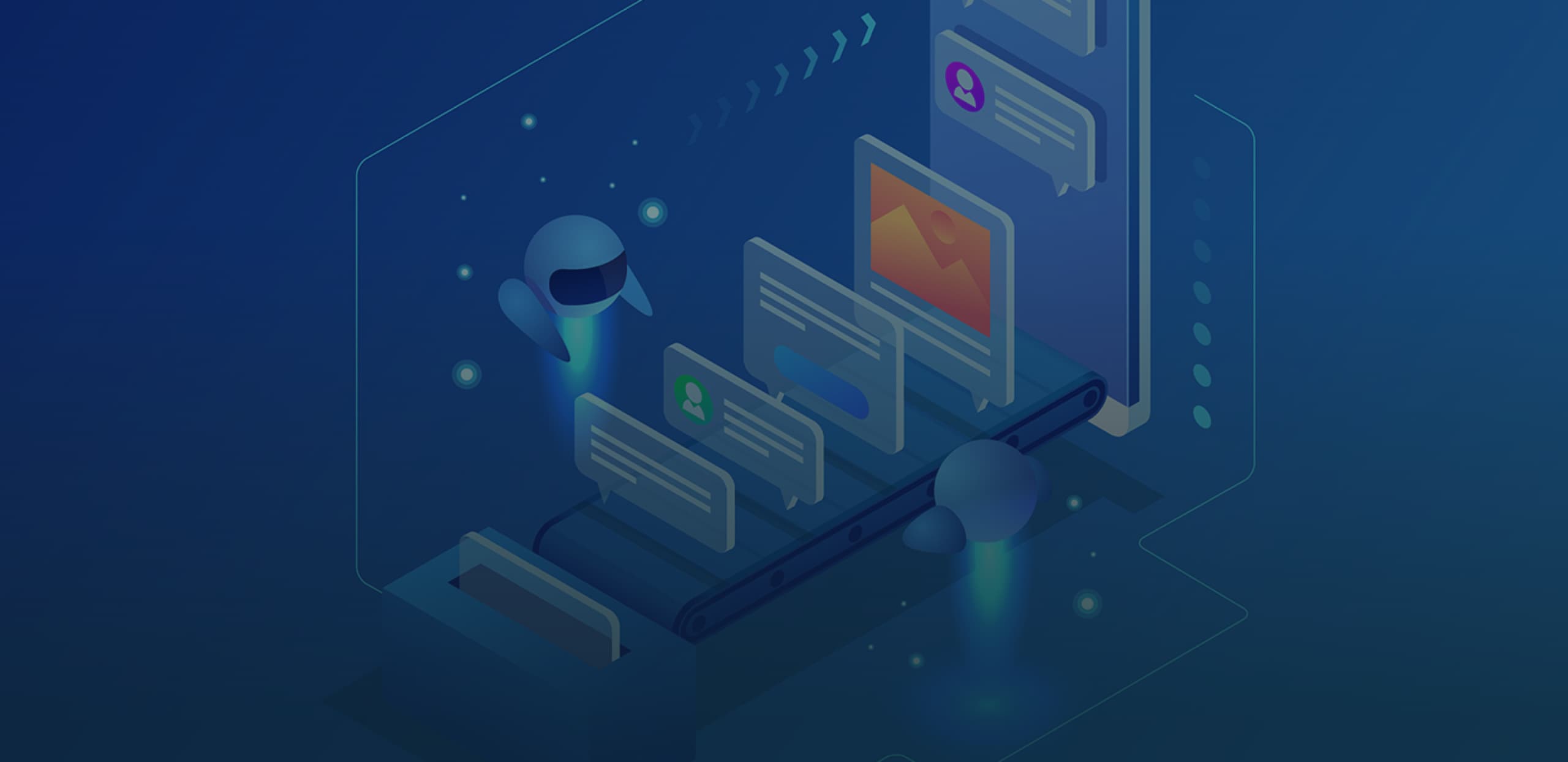
AI In Mobile
Heinz Riehl Chair Professor of Business,
Professor of Technology, Operations,
and Statistics, NYU Stern School,
Professor of Marketing,
NYU Stern School &
Director of Masters in Business Analytics,
NYU Stern.
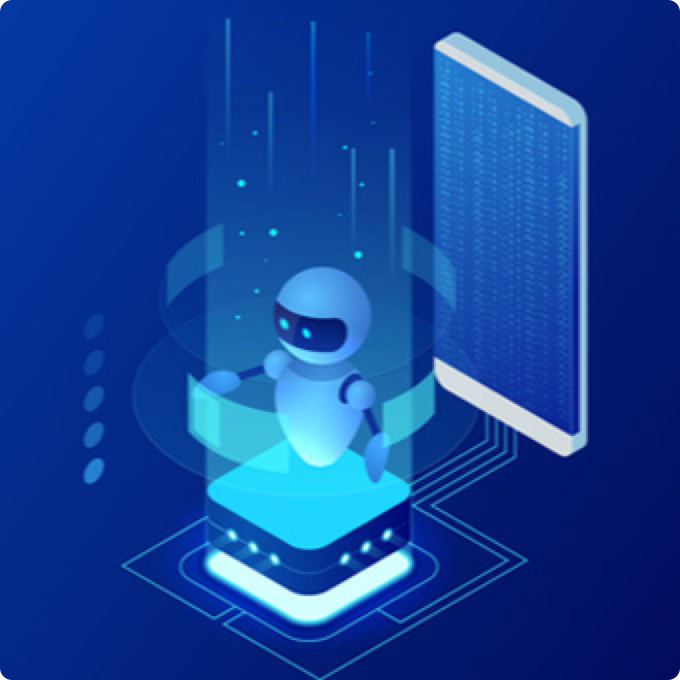
Summary
An average individual looks at his/her phone for more than 2.5 hours and makes about 35,000 decisions in a single dayi. Smartphones have led to the emergence of new consumer behavior, turning the spotlight on Artificial Intelligence.
With companies aggressively pursuing the benefits of an AI-driven future, maximizing the power of AI from our mobile phones is predicted to increase dramatically. AI is making great inroads into our lives, from virtual assistants like Siri and Alexa to voice driven Apps to intelligent camera, the possibilities are hard to ignore. Next-generation mobile experiences hold great promise for both brands and consumers.
In an innovation-focused digital age, Machine Learning algorithms are more than a fad; they serve as a catalyst for creating meaningful customer experiences.
Prashant Vaishnav, AVP & Senior Director, Sales, EdgeVerve, discussed the Future of AI in mobile technology with Professor Anindya Ghose.
I think the best way to answer that question would be first to explore what an AI algorithm does, and then we can situate it in the mobile context. Whether it’s a supervised or unsupervised Machine Learning algorithm, an AI algorithm is essentially one that is trying to best imitate or mimic human behavior. So, the fundamental property in the AI algorithm is really a data-hungry algorithm, in the sense that the more data you feed into it, the better it’s able to learn, set up, improvise, adapt, and imitate human behavior. So now, how does this all fit in the mobile context?
Mobile phones, are now unequivocally considered the single biggest source of consumer behavior data. We are logging in 150 times on an average on our phone; in other words, we are spending about five to six hours every day on the phone, and this is just pre-COVID levels; post-COVID, it’s even higher. Every interaction we have with our phone, whether it’s a click or a slide or any other interaction with the device, gets captured by different sorts of data providers and companies. And what that interaction is creating is this massive goldmine of data that is incredibly useful for various brands, marketers, advertisers, and companies to understand better what the user or consumer is really like. The better they understand these users, the more they can do something about reciprocity-based interaction.
Whether it’s in the form of a curated or targeted ad or a highly personalized pricing discount, various economic incentives can be aligned once they delve deeper into the data. That’s the big picture here, how can AI in a mobile form be used effectively to understand the specific user better and then act upon that data.
So, this is a topic that deeply interests me. A couple of years back, I wrote a book on this topic called TAP: Unlocking the Mobile Economy. It got a lot of attention all over the world primarily because we were able to produce several case studies, from both my consulting work and academic research, that showcase the various effects mobile phones can have on consumers. In summary, what you found is that nine forces are shaping the mobile economy by directly shaping consumer behavior.
Going back to the first part of the question, what has fundamentally changed, is that consumers are even more deeply embedded on their smartphones. Smartphone has become sort of a remote control of their life. Every meaningful aspect of their life has been influenced by an app or some other feature of the phone. And because we’re spending way more time on the phone, we are generating lots of data, which is now available to train the algorithms to better understand what this person is thinking.
I would say the fascinating thing about this is that we’ve had the opportunity to work very closely with a large number of different companies across countries. Apart from working in the US, I have traveled to Europe, South Korea, and China.
Certain industries lead this frontier; if I had to rank the top three, I would say that they are retail/E-commerce, travel (hospitality), and media. In my view, those would be the dominant ones, but there are also others catching up, like retail banking. We’ve seen some interesting examples in the CPG world. It is only a matter of time before we see more and more influx of these companies adopting AI-based solutions for the mobile economy.
One of the most fascinating use cases we worked on was for one of the largest shopping malls in Asia. This was a few years back when we came up with the idea of trajectory-based mobile targeting, which quickly became a favorite for many brands. Let’s say somebody is standing in front of Starbucks and, the Starbucks app might send them an offer about a 20% discount or a ‘buy one, get one free’ message. Coffee is a relatively inexpensive product, right? For a couple of bucks, you can get coffee. Let’s pick a more expensive product like an Apple product or an electronic device. Now consider a shopping mall with an Apple store and its competitor stores like Samsung, Xiaomi, and Huawei. Now, if you’re only using location-based targeting, then a customer who’s standing in front of an Apple store is going to generate some false positives. In other words, I might be standing in front of an Apple store, but that’s not because I have an interest in Apple products, but it’s because my friend decided that we would meet in front of the Apple store. And when the two of us converge, we’ll go and grab a coffee.
In this case, the Apple store was simply a landmark for two individuals to meet in person. That’s an example of a false positive because neither consumer was interested in any Apple products. That’s where location-based targeting fails. We proposed to the shopping mall that instead of using just real-time location, we would use the previous 20-30 mins trajectory of each customer within the shopping mall to examine the other stores they visited before arriving at any spot. They will then be able to feed that data into an AI algorithm and recommend certain products.
So that’s what we proposed. And it was a spectacular success.
We were able to essentially use the trajectory data of several hundred thousand customers over several weeks in these malls and propose this first-of-its-kind recommender system. Recommender systems are widely used in the online world like Netflix, Amazon, and Spotify. We were the first to build this in the offline world where we said, people who go to the Samsung, Google, and Huawei store are interested in a specific product category. Therefore, we should offer them a personalized discount from these categories only from a competing store like Apple.
For example, if a person purchases/searches a book on Amazon, the algorithm predicts the person’s next purchase and recommends products based on it. Netflix, too, is powered by AI algorithms, helping personalize customer experiences. We were the first to contextualize and execute this in the offline world.
In hindsight, I think there were many pitfalls along the way. It wasn’t a smooth journey, but we learned through our failures. But once we executed that, we saw spectacular success since there’s a lot more data that can be tapped from the customer’s prior trajectory. Since then, the algorithms have been widely used in several other environments. We have also been working with airports to map the trajectory of transit passengers when they go from one duty-free store to another and even within the store. It’s exciting to see our methods and algorithms having such a wide applicability.
Loved what you read?
Get practical thought leadership articles on AI and Automation delivered to your inbox
Loved what you read?
Get practical thought leadership articles on AI and Automation delivered to your inbox
Over the last two decades, I’ve worked with a bunch of telecom companies in precisely monetizing this sort of data. Some of the earlier work was with South Korea Telecom, China mobile, and Korea Telecom. Then we worked with Telefonica in Germany and then of late I have consulted for Verizon here in the US. So, to take a step back, while there is this thinking that the digital platforms are the only source of very rich consumer data, my own experience suggests that the telecom companies and retailers, in many cases, may often have even richer data than the digital platforms. It turns out that in many cases, the telecom companies and retailers have far better data than what just a digital platform will have. That’s primarily because they can leverage every single interaction that the consumer has with the phone and through some strategic mergers and acquisitions can often stitch the data across silos.
I’ll give you a precise example. About four years back, I was involved as an expert witness in a large litigation involving the merger acquisition between a telecom provider and a major tech firm. Specifically, this was the lawsuit involving the acquisition of AOL by Verizon. During this litigation process, I understood how valuable their data was and how rich and granular it was.
Advertisers today have many choices among telecom companies, broadcast TV and non-linear TV providers, social network platforms, digital news media sites and retailer media networks to use consumer-level data for targeted advertising. Aside from these, you have isolated pockets of data from media agencies who are buying data brokers and from the consulting companies now essentially transforming them into the large media agencies.
There are a lot of different data sources that a brand can tap into for advertising. This means that the advertisers have a lot of choices with whom they want to collaborate with. I think it’s going to be really exciting. In the next five years or so, you’re going to see more and more disruption happening in this space and more companies having access to similar consumer data. So, there’s going to be more competition in this space.
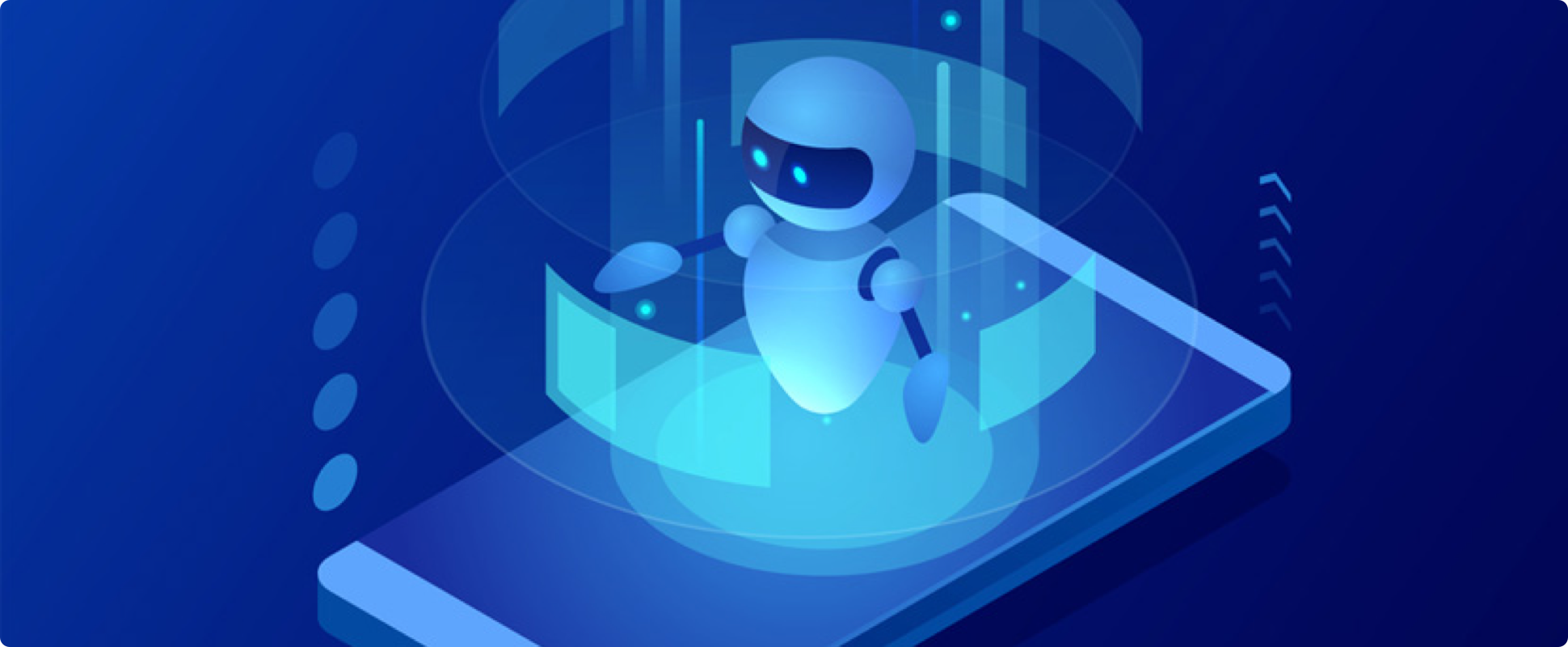
Having worked with large brands across the globe, I firmly believe that most organizations really do not have a vested interest or an incentive to abuse customer data intentionally. What I mean by that is, we live in an adversarial world where no matter, who you are, you always have bad actors (hackers, security breachers etc.) on the other side. The bigger, the organization becomes, the more likely it will face the attention of adversarial actors. The good news is that most organizations understand that this is a concept. I talked about it in my book that they need to act like a Butler and not a Stalker, meaning that their access to your data will give them unprecedented opportunities to get to know you. However, they need to act like your concierge similar to the one in a hotel. They should not make the mistake of acting like a stalker because there’s a very thin line dividing what is cool vs. what is creepy.
I’ve been working on monetizing location data; I’ve also been very interested in using Machine Learning and AI to build algorithms to protect our data. The intent is to make it a win-win for both sides. One of our most recent research projects got awarded in a globally recognized conference last December in Germany. We were able to build a method that for the first time showed how companies can use less data and yet get higher ROI.
We showed that for a given individual using our Machine Learning algorithms, you can use, let’s say 20% less data, and yet get 10% more effectiveness for your offers, ads and, coupons. We’re now planning to patent those methods and algorithms, and then eventually we’ll commercialize it, and hopefully, that’ll get attention in the market. The good news is, it’s possible for AI to become your butler and concierge and not a stalker.
In the present, the answer to that would vary based on which geography or geographical location you’re in. Having worked with companies in Asia, Europe and the Americas, and having tried to understand consumer behavior, it’s evident that there are major differences across geographies.
An example of this is when it comes to consumers adopting new technologies or new features, or new phenomena. The one country that’s always ahead of the curve is China. Customers in China are more adaptable or flexible when it comes to giving a new product or any service a try without overtly being concerned about the outcome or what’s happening with their data. It’s an interesting feature of the digital economy there because of the fact that customers are willing to quickly adopt new phenomena, it also creates a positive environment for entrepreneurs to generate start-ups in order to monetize that phenomena. After all, they know that they’ll have a strong customer base that they can monetize and can tap into.
The converse is also true in Europe, where, despite having pockets of excellence in AI, we do not see as many tech starts up emerging. For instance, consider Sweden, or parts of France, where there’s a lot of AI expertise. However, they are unable to make any breakthroughs in monetizing this confluence of AI and mobile economy because of strict regulatory constraints from the European Commission and associated regulators. Outside of the likes of Spotify, Zalando, and ASOS, we are not going to find many tech behemoths that have come out of Europe, in the last 30-40 years. Conversely, if you look at China, US, S. Korea, or even Israel, you see a number of fascinating examples of tech entrepreneurship.
I think India is very much in the middle. We have not seen too restrictive constraints from regulators in India. They have stepped in occasionally, but they haven’t imposed the kind of regulations that handicap companies, like the European Commission. For instance, GDPR, in my opinion, is really problematic because it imposes constraints on what companies can do, and what is technically feasible, and one can see the effects of that sort of regulation. So, it ends up having exactly the same effects that the regulators did not want, and this has happened largely due to the imposition of complex constraints on what companies can do with consumer data.
The idea of bringing regulators and companies on the same table to have a common understanding and etch out a sustainable and feasible AI strategy, is going to be the biggest challenge in the coming years in the world of AI. In this respect, India needs to rapidly formulate a concrete AI strategy in order to compete with China and USA.
Disclaimer The opinions expressed within this article are the personal opinions of the author. Any references made with respect to any company or person form a part of the author’s opinion. The facts and opinions appearing in the article do not reflect the views of Edgeverve or its Affiliates and Edgeverve does not assume any responsibility or liability for the same.
PREVIOUS ARTICLE
NEXT ARTICLE
PREVIOUS ARTICLE
NEXT ARTICLE