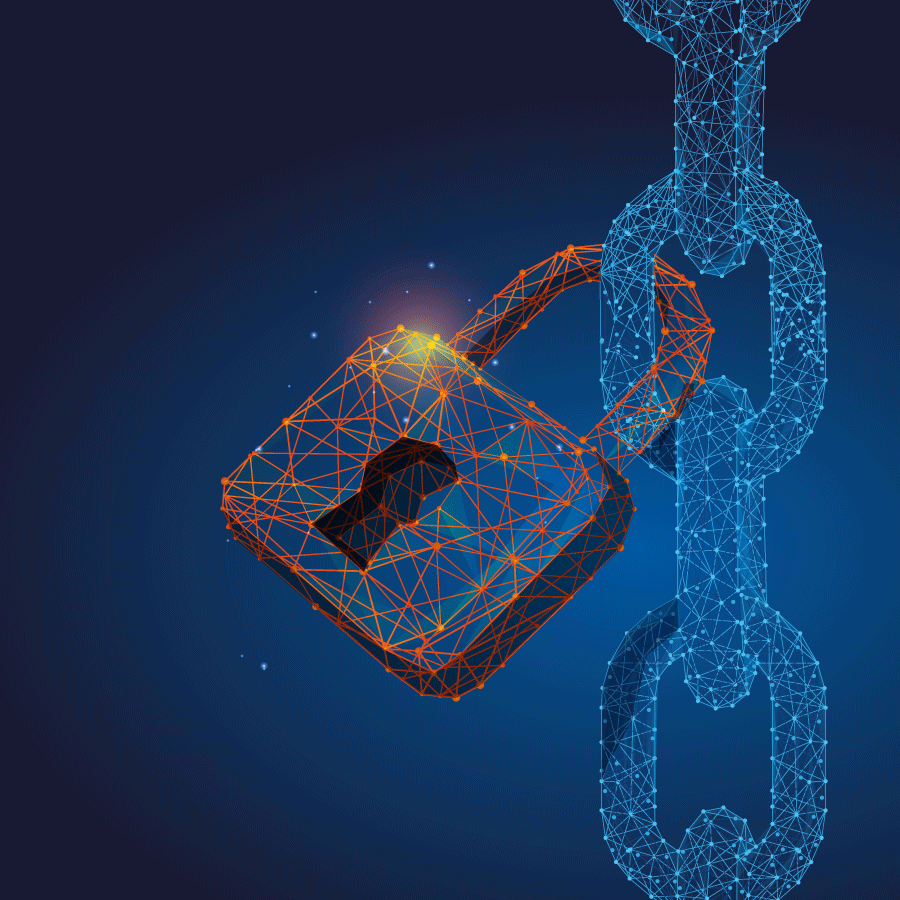
In an era marked by rapid growth, innovation, and endless ambition, the current crisis has created a need for a sharp focus on the things that matter. Enterprises must now be resilient, innovative, and efficient while staying ahead of the competition. The proliferation of technology, specifically intelligent technologies, is set to play an increasingly important role in this endeavor. We are at a juncture where the abilities of machines far exceed productivity goals, impacting even planning and decision-making processes. Our current experience of the need for robust technology means that it won’t be long before cognitive technologies permeate every aspect of human life from entertainment and healthcare to transport and, perhaps, even space travel. An average person’s life is already so intertwined with technology that they trust machines to help them navigate to a destination, report their vitals, and offer lifestyle recommendations.
For enterprises, this change is even more significant. Across departments, an increasing number of companies will automate business processes alongside a larger volume of decision-making. AI and ML, emerging technologies until recently, are now firmly in the mainstream and will drive the future of enterprise growth. The growth figures are a testament to AI’s popularity with a report1 from market research firm Fortune Business Insights stating that the global AI market is set to touch USD 202.57 billion by 2026, up from just USD 20.67 billion in 2018 at a CAGR of 33.1%. Procurement, sales, marketing, production, finance, human resources, regulation, and compliance are just some examples of departments seeing the transformative effects of AI-based technology. It is an exciting phase. These AI and Automation based technologies will augment human productivity, reduce average running costs, and all but eliminate the need for manual intervention in deterministic tasks, creating an environment of agility and continuous innovation. Adoption and implementation, however, are not the only areas for enterprises to consider.
A Question of Safety :
As enterprise dependency on machine-driven cognitive capabilities increases, it is pertinent to explore AI system security. Are AI systems tamper-proof? How can they be made secure? From a more fundamental standpoint, can the prevailing narrative of security and control, adequately address the potentially unique threats that AI-based systems can pose? To answer these questions, we need to understand the broad themes that determine the resilience and effectiveness of AI implementations. These include:
- The vulnerabilities of AI systems and disruptions
- AI’s value drivers and how they can be protected from malicious actors
- The unknown threat actors and attack surface for AI systems
- The differences between traditional security controls and those of AI systems
Before we delve into these topics, it may be pertinent to understand an AI system’s life cycle.
Stages of AI Lifecycle :
AI systems have two stages in their life cycle – learning and inference. In the learning phase, a model is trained using available data. The data can either be labeled (supervised learning) or unlabelled (unsupervised learning). In the inference phase, as the name suggests, a model makes inferences based on the framework developed in the learning phase. Systems can also learn from their inferences actively through a process known as reinforcement learning. Each of these stages carries distinct security threats. Let’s understand them better.
A Safe Journey Ahead
The strength of AI’s value proposition means that its proliferation is inevitable, irrespective of temporary concerns from security professionals, regulatory authorities, privacy advocates, system auditors, or even the legal fraternity. Resistance to adoption would be imprudent, if not downright foolish. Moving forward requires preparedness that understands the risks and looks to mitigate them. Data protection must move beyond a mindset of purely legal considerations or GDPR compliances to one that safeguards the most valuable resource we know. Companies should develop data protection and access policies that offer extreme visibility into data use, especially at the learning stages. Enterprises that implement the necessary controls, checks, and balances will enjoy the benefits of a secure AI system that generates valuable, explainable, and justifiable outcomes.