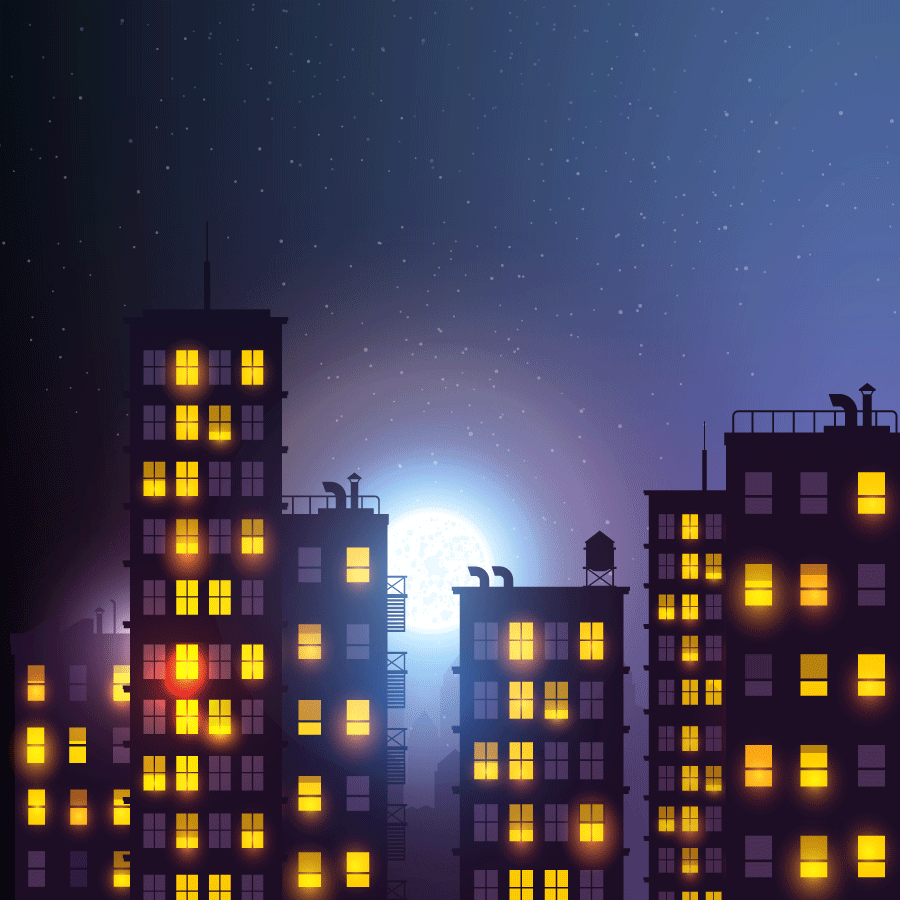
Covid-19 pandemic is a disruptive event like no other and has impacted many critical enterprise business operations worldwide. Global enterprises capitalize on technology to better manage their business. IT operations are the operational layer of an enterprise’s technology landscape. However, enterprises are prone to disruption as demand, supply, and performance flounder. In this article, the experts share their perspective on how enterprises can future-proof IT operations by adopting AIOps to manage the technology landscape intuitively and avoid disruption. Enterprises use digital technology to integrate applications, manage a massive volume of data, and streamline processes. IT operations constitute the heavy lifting by the IT team across the technology landscape. Business enterprises function with clockwork precision and efficiency when IT operations are seamless and have the ability to scale up without interruption.
To put IT operations in perspective, let us understand how a power utility provides 24/7/365 electricity. The utility needs to align demand for power with power supply. The grid needs to optimize the capacity to manage spikes in consumption. The power utility also needs to provide consumers with a choice to switch between conventional and renewable sources of energy. A digital utility uses a smart grid to ‘keep the lights on’ always. The bedrock of the digital utility is operational excellence in IT operations.
The smart grid automates the operational aspects of IT to provide a reliable power supply. A digital utility incorporates artificial intelligence (AI) to provide forensic intelligence for managing IT operations. AIOps leverages machine learning and data science to ingest events and metrics from IT systems across the business, while identifying anomalies, correlating events, and taking preventive action to avoid disruption.
Essentially, AIOps applies AI to IT operations so that enterprises can smartly run IT and scale up the business. AIOps tools identify early symptoms of IT dysfunction, find issues that have occurred in the past to accelerate resolution, and address system bottlenecks across the IT value chain.
AI in IT operations improves predictability by leveraging past knowledge for the future, thereby ensuring reliability in business operations. In real-time monitoring, the availability of dedicated AI computed resources allow IT to become increasingly agile and responsive in near real-time.
During the global lockdown in the aftermath of the COVID-19 pandemic, AIOps pre-empts the disruption of critical IT systems. By capitalizing on machine learning and data interpretation capabilities, AIOps reduces human intervention while undertaking automated troubleshooting / investigations.
Beginning with AIOps – Breaking down silos
AIOps can streamline and enhance company-wide IT functions, reduce repetitive labor and create new and better processes through data-led insight. While a lot of AIOps products in the market talk about applying AI to a few specific IT cases, AI can be applied in any use case where past data is available. Some of the roles, as defined by ITIL, that could easily and effectively reap the benefits of AIOps include service operations teams like incident management, L1 and L2 support teams, request fulfillment teams, access managers, IT operations control teams, and application management teams. Continual services improvement teams such as process owners and process architects, can do a lot with AIOps
Service design teams like catalogue management, service level management, risk management, capacity and availability management, and compliance team members, can also find great advantages with AIOps.
To elaborate on this further, we’ve listed below seven characteristics that define a true AIOps solution, as mentioned by analysts at Ovum (Ondia). These were featured in a recent eBook1 created by EdgeVerve in collaboration with AIBusiness.