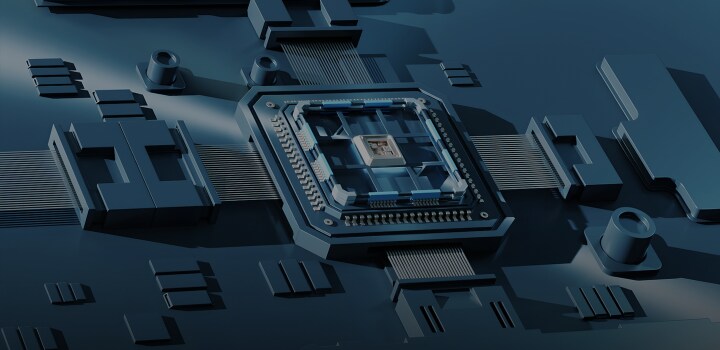
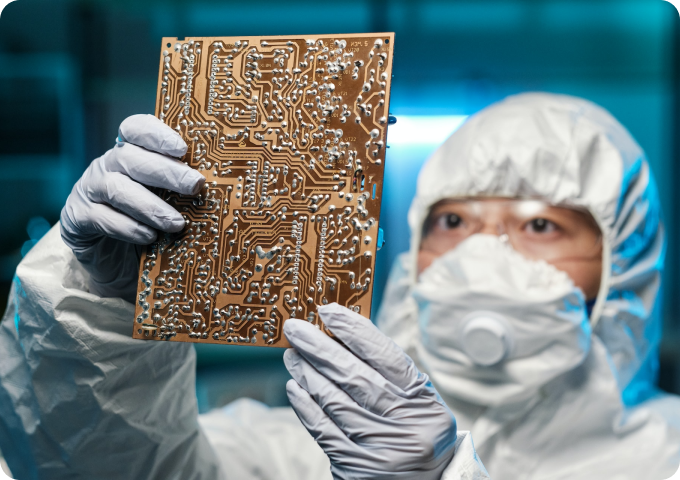
The semiconductor industry has the potential to become a trillion-dollar industry. Despite this, several challenges must be overcome, including legacy processes, long setup timelines, and a shortage of skilled labourers. One way to address these challenges is by leveraging generative AI. The technology can help shorten production cycles, optimize costs and resources, and increase innovation velocity, which is the need of the hour for the semiconductor industry. Learn how Generative AI can deliver higher value to this industry.
The global semiconductor industry is sitting on a goldmine of growth with prospects of becoming a trillion-dollar industry by 20301. In the US alone, the enactment of the CHIPS and Science Act in August 2022 has seen a mammoth $210 billion in private investment announcements across 19 States. The domestic semiconductor industry in the US is also set to receive $76 billion in subsidies2. Semiconductor companies like TSMC plan to triple their investment3 to build manufacturing facilities and hire aggressively to address the increasing demand for chips and plug the demand and supply gap. Not to be left behind, the European Union is planning to invest €43 Bn4 to double the bloc’s share of global chip output. These figures leave no room for doubt that we will witness massive growth in this domain in the US and globally.
While growth is happening in all aspects of the semiconductor industry (See Box), in this article, we will explore what the next few years have in store for semiconductor fabs in the US. Essentially, US-based semiconductor manufacturers need to focus on how to set the proper foundation for the future. And finally, the role generative AI will play in this journey.
The new foundries for the future will have a Halo Effect on the entire semiconductor value chain. As foundries build efficiencies and agility with new technology, the designers, fabless semiconductor firms, integrated device manufacturers, and other ecosystem players will also benefit. Collectively the ecosystem will be able to move away from the inertia of manual processes and synergize better outcomes. For instance, generative AI may lower the barrier for complex chip designers by giving design options to choose from or codifying best practices to build, for instance, an amplifier. Synopsys5, for example, is working on design space optimization (DSO) that can autonomously search design spaces for optimal solutions enabling massive scaling in the exploration of choices and automating less consequential decisions.
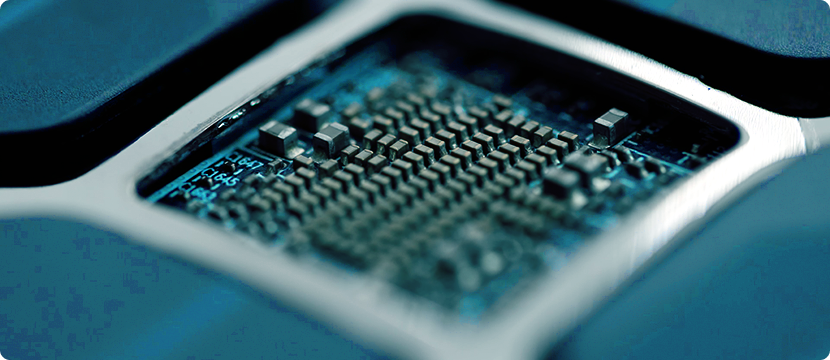
Legacy Challenges Playing Spoilsport
A trillion-dollar semiconductor industry is terrific news, but this silver lining comes with a few spots of grey that need ironing out. The elephant in the room is the traditional chip manufacturing approach, which must scale to deliver for the future. The breakneck speed of technology evolution for all things that consumers demand – heavily dependent on the semiconductor industry – needs constant innovation, high quality, and rapid time to market with new products and materials. Yet, existing systems and processes need help to keep pace with increasing design complexity and reducing chip sizes leading to rising costs and defect rates.
Intel predicts that by 2030, microchips will have ~10x more transistors or one trillion transistors per chip6. Designing and fabricating these complex chips with increased functionality, diverse applications, and heterogenous architectures will not be child’s play. At least not with the way things are at present. Several challenges are putting the brakes on players’ ability to aggressively exploit the semiconductor industry’s growth potential. Some of these include:
- Long timelines for setup – A five-year timeline7 for setting up the semiconductor fab is quite long and needs to be optimized.
- A two-fold talent shortage – According to McKinsey, the industry faces a shortage of skilled labourers8 to build the massive semiconductors fabs and a lack of trained personnel9 to operate them once built.
- Complex, legacy processes – A typical chip-making process has hundreds of steps10, and the time frame from design to mass production can be months. One of the reasons for this is the tremendous amount of human intervention involved in this process.
One might wonder why companies that plan to spend billions of dollars building semiconductor fabs still use a legacy approach toward chip manufacturing. The answer lies in human psychology. Key decision-makers ask themselves, “Why to fix a process that has worked for more than four decades?”
The existing semiconductor fabs have been around, unchanged or with very few minor tweaks, for decades because no one wants to risk a breakdown, which is a fair point. However, now that there is a new opportunity and billions of dollars are being invested in new factories, semiconductor players need to ask themselves some hard questions:
- Are the new semiconductor fabs being built right? How should we design these factories to be relevant in the coming decades?
- How do we ensure that legacy challenges and decades of inefficiencies are not inherited?
- What new capabilities can we deploy to improve ROI, yield, operational efficiency index (OEI), and other KPIs?
- How do we get the chips to market faster and with better capabilities?
- How do we use technology to shorten production cycles, optimize costs and resources, and increase innovation velocity?
Finding the correct answers to these hard questions will certainly lead to billions in cost savings and process improvements. Hence it is worth asking and answering these questions correctly. One of the potential answers to these questions could be our ability to leverage artificial intelligence, especially generative AI, and how it can drive cognitive operations and amplify the human potential for exceptional gains.
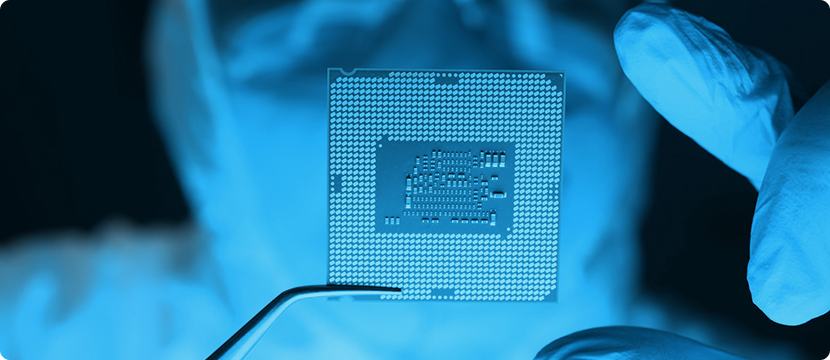
Understanding Generative AI
We did an exciting experiment with ChatGPT 4 and asked the model to explain generative AI. After several prompts and deeper probes, it came up with this
Large Language Models (LLMs) are a type of generative AI model specifically designed for natural language processing (NLP) tasks. They are trained on vast amounts of text data, enabling them to understand and generate human-like text based on the context provided. LLMs can be used for various purposes, such as text completion, translation, summarization, and content generation.
OpenAI has developed a series of LLMs called Generative Pre-Trained Transformers (GPT), while Google has built two LLMs for NLP: LaMDA (Language Model for Dialog Applications) and PaLM (Pathways Language Model). These models can be fine-tuned for specific tasks, making them highly versatile and suitable for various applications. For example, ChatGPT and Bard are chatbots based on GPT 4 and LaMDA, respectively.
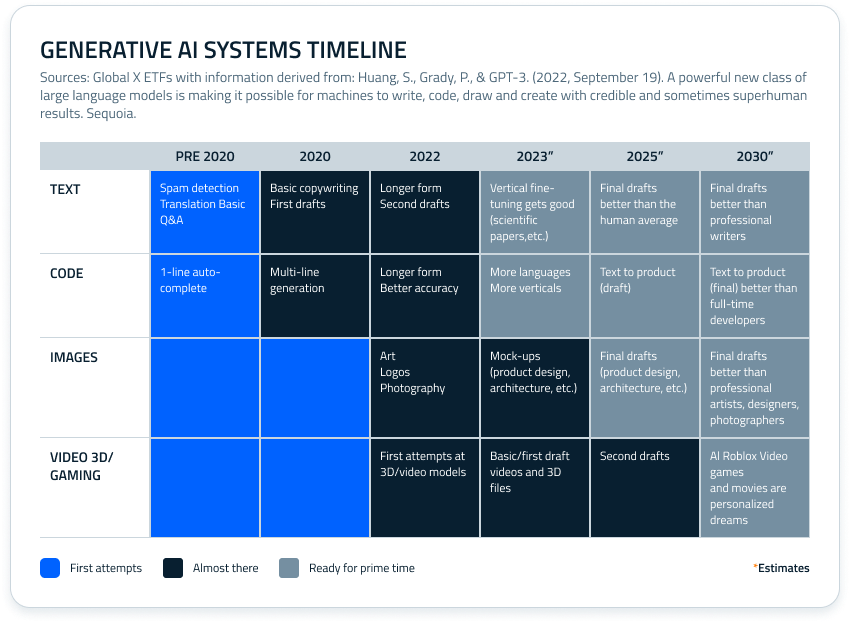
Generative AI as the Agent for Change
Artificial Intelligence is not new in production. Audi, for instance, has been using AI11 to automate quality testing, detecting flaws in components and the quality of spot welds, etc. More recently, they have also deployed a generative AI model called felGAN12 to inspire new rim design ideas for wheels.
The recent interest in generative AI models like ChatGPT has brought the immense potential of Large Language Models into the limelight. Generative AI can be the knight in shining armour that can help semiconductor companies break free from the shackles of tradition and outdated approaches toward manufacturing. It can help optimize the manufacturing process itself and, along the way, help augment the capabilities and performance of the humans in the process. Some potential use cases of how it can help set the foundation of a new era of semiconductor fabs include:
- Improve human decision-making in setting up new machinery: One way to address long fab setup times is to help people make better decisions. People involved in setting up the new factories may need to learn all the challenges or best practices that affect yield, quality, etc. Generative AI models can ingest past data to answer queries or generate a set of instructions to double-check everything. Even small changes can have a significant impact on an industry like semiconductors. For instance, minimizing the number of setups needed in the new factory or minimizing human error can save millions of dollars. Building a fab on a specific set of quality standards only to realize that the standards were different is a costly error that can take tens of millions of dollars to correct. Similarly, it can; for example, help choose the best design for an amplifier or a microcontroller from multiple options based on several parameters.
- Optimize chip design for manufacturing: Today, Infosys experts estimate that nearly 30-40% of the time in chip design is wasted due to process inefficiencies. Does the design to tapeout process need hundreds of steps? With Generative AI, it’s possible to cut out unnecessary steps and automate the design process13. Generative design can minimize human input and overcome the limitations of a traditional Electronics design to better align with Process Design Kits (PDKs). Semiconductor players can also use reinforcement learning to optimize the component placement, reducing product-development life cycle time14 from weeks to hours. Fewer steps with automated decisions also translate to higher yields at a much lower cost while addressing the talent gap.
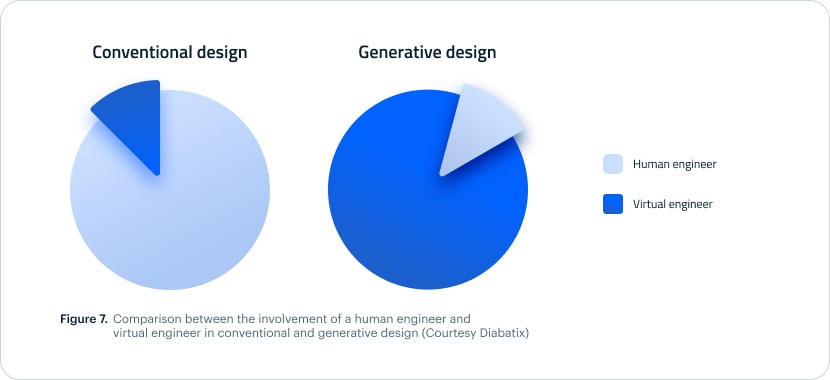
- Optimize scheduling and resource planning: Most work done on-site in semiconductor plants is still dependent on manual decision-making, which is often time-consuming, limited in scope, and prone to errors. Generative scheduling15 can help create multiple scheduling and resource allocation scenarios and compare them in real-time to improve critical decisions regarding labour, equipment, installation rates, access, start-up sequence, etc. This end-to-end optimized plan can help minimize cost and schedule overruns.
- Improve metrology and defect detection: Shrinking device sizes with billions of components working together in tight specifications at an atomic level16 have made metrology an essential process in chip manufacturing. Even the smallest of changes can render a chip unusable. While the quality specifications get more complex, the inspections remain manual – a scenario not viable for the new foundries. Generative AI can leverage unsupervised learning to detect defects, generating realistic images of defective chips to empower visual inspection and improve anomaly detection.
- Enable straight-through processing with intelligent transactions: Business process transformation has mostly been around functionality. While that is needed, the ultimate process efficiency gains come from straight-through processing (STP). To enable STP, the systems must be able to process and consume both structured and unstructured data. AI can help manage all structured and unstructured data in the semiconductor fab, bringing information from various sources, unifying it, and delivering a single source of truth, to connecting operational areas such as orders, inventory, logistics, supply chain, and more. This facilitates Straight Through Processing to speed up transaction processing times, improve compliance, manage risks, and amplify user experience.
- Reduce the cost and impact of indirect labour: Two of the core metrics of a fab – capacity and time to volume – depend not only on the manufacturing process but also on indirect work such as building the factory, plumbing, contracting, etc. According to McKinsey, indirect labour accounts for ~18-20%16 of annual manufacturing expenses. Given the talent shortage and the associated costs, the industry must look at digital solutions. Automating processes, extracting document data, generating insights, etc., can significantly impact contract management and negotiations, risk management, safety and quality, preventive maintenance, etc. Companies can augment human decision-making and accelerate value by enabling NLP queries with generative AI.
Loved what you read?
Get practical thought leadership articles on AI and Automation delivered to your inbox
Loved what you read?
Get practical thought leadership articles on AI and Automation delivered to your inbox
Towards a Smarter Future
Generative AI is just the first chapter in the book of semiconductor chip manufacturing. The time isn’t far when we will see truly smart semiconductor fabs where the manufacturing processes work in pitch-perfect synergy to improve yields and optimize costs. At the same time, with every manufacturing component sharing information, water usage, emissions, and power consumption can be tracked in real time. This will enable ESG-centric (Environment, Society, Governance) decision-making to help the semiconductor industry meet the standards of the most demanding regulatory frameworks.
Descriptive, prescriptive, and predictive AI-driven technologies will help build future-proofed semiconductor fabs that can mine gold from the goldmine of the opportunity presented to them. To learn more about how you can build future-proof AI-enable factories, write to us at edge@edgeverve.com
Disclaimer Any opinions, findings, and conclusions or recommendations expressed in this material are those of the author(s) and do not necessarily reflect the views of the respective institutions or funding agencies
References:
- https://www.mckinsey.com/industries/semiconductors/our-insights/the-semiconductor-decade-a-trillion-dollar-industry
- https://www.google.com/search?q=US+government+76+billion+investment+in+semi+conductor&rlz=1C1JZAP_enIN832IN832&oq=US+government+76+billion+investment+in+semi+conductor&aqs=chrome..69i57j33i10i160l3.10311j0j4&sourceid=chrome&ie=UTF-8
- https://www.nytimes.com/2022/12/06/technology/tsmc-chips-factory-phoenix.html
- https://www.reuters.com/technology/eu-countries-lawmakers-likely-clinch-deal-chips-act-april-18-sources-2023-04-05/
- https://blogs.synopsys.com/from-silicon-to-software/2021/07/15/ai-chip-design-process/
- https://www.pcgamer.com/intel-says-there-will-be-one-trillion-transistors-on-chips-by-2030/
- https://www.marketplace.org/2022/08/23/what-does-it-take-for-chip-manufacturers-to-get-a-new-plant-up-and-running/
- https://www.mckinsey.com/industries/industrials-and-electronics/our-insights/semiconductor-fabs-construction-challenges-in-the-united-states
- https://www.axios.com/2022/03/23/chip-makers-labor-market-squeeze
- https://www.asml.com/en/technology/all-about-microchips/how-microchips-are-made
- https://www.audi-mediacenter.com/en/press-releases/smart-production-how-audi-is-designing-the-production-of-the-future-14786
- https://www.metal-am.com/articles/optimised-thermal-management-in-semiconductor-fabrication-using-ai-enabled-generative-design-and-additive-manufacturing/
- https://www.gartner.com/en/articles/beyond-chatgpt-the-future-of-generative-ai-for-enterprises
- https://www.mckinsey.com/capabilities/operations/our-insights/generative-scheduling-saving-time-and-money-in-capital-projects
- https://www.ncbi.nlm.nih.gov/pmc/articles/PMC6605074/
- https://www.mckinsey.com/industries/semiconductors/our-insights/reducing-indirect-labor-costs-at-semiconductor-companies