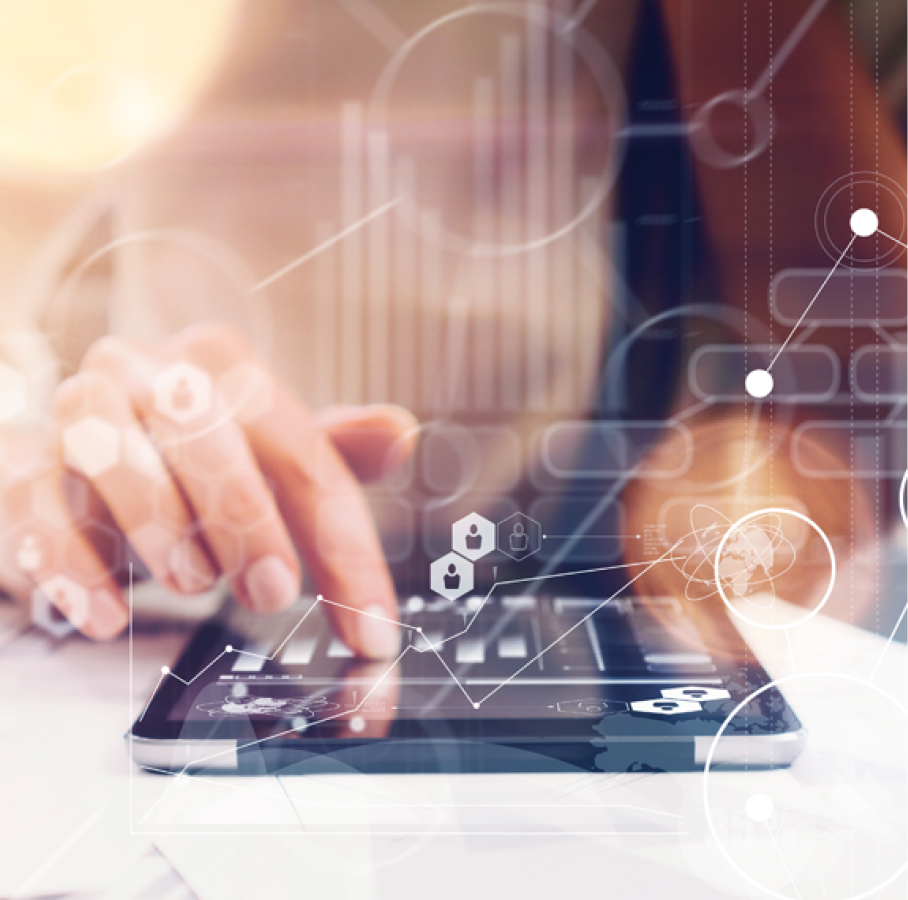
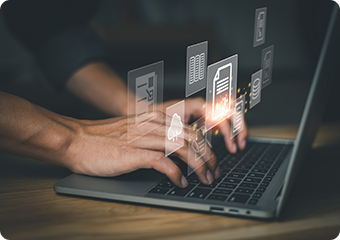
Today, organizations wield AI for document processing in much the same way that 18th-century miners used hydraulic pressure to extract gold from rock. By creating a document processing pipeline, advanced AI platforms use a combination of technologies from NLP to OCR to ‘wash away’ the fluff and extract granular and macro insights from raw, often unstructured, document data. How can your enterprise turn this cost centre of digitization into a gold mine of information? Read on to know how.
In 1849, when the California Gold Rush was beginning to peak, independent and itinerant miners still used panning techniques to sift river gravel for nuggets of gold. But as mining consortiums replaced individual miners, it became evident that panning was unlikely to yield a profit at scale. Instead, companies began excavating tons of gold-bearing rock and used large high-pressure water hoses to blast the impurities from the ore.
Similarly, in industries like insurance, banking, logistics, and even telecom, where core processes still involve physical paperwork, Intelligent Document Processing (IDP) can fast-track data digitization and save hundreds of thousands of person-hours every month.
Part of the reason is a technology-first approach to IDP implementation that fails to consider end-user needs and business value creation. Customers often equate IDP with optical character recognition (OCR), assuming that the results should drive consistent business value as long as the tech works as intended. By taking this stance, enterprise leaders set themselves up for failure and effectively deny themselves the opportunity to maximize returns on their IDP investments.
While undoubtedly concerned with extracting data from documents, the IDP process aims to convert data to structured information that can be contextualized to various business use cases. And what makes IDP different from run-of-the-mill AI deployments is the sheer complexity and variations involved with document-based data extraction.
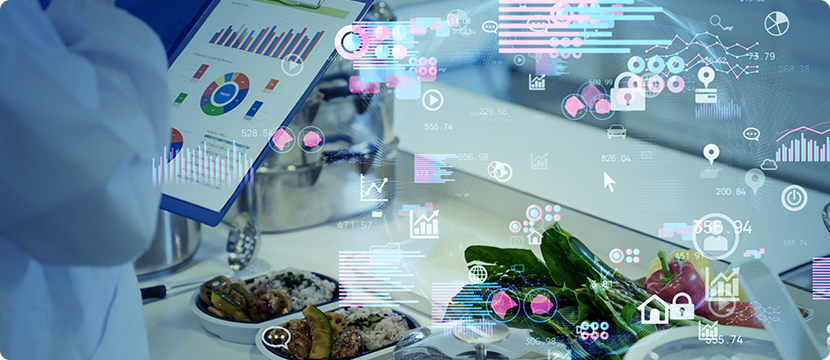
Industries and even individual enterprises mature and grow at different rates. Therefore, part of the challenge in implementing IDP is building a data extraction and contextualization system that is flexible enough to address evolving business cases, especially in disrupted and emerging markets.
Complicating matters are the hundreds of variations within the spectrum of enterprise documentation.
In addition, each document’s layout can differ widely, with some documents laid out in a top-to-bottom schema while others have data laid out horizontally. Mining data from all these elements mean deploying a bevy of technologies and techniques, including NLP, deep language learning, OCR, AI analytics, and neural networks.
Since much document-based data is unstructured, training IDP data models can feel like a shot in the dark. In many cases, the initial sampling strategy has a disproportionate impact on the success of the project. For instance, a significant shipping consultancy company limited the scope of their IDP project to english-only PDF purchase orders. In reality, the company received POs in multiple languages, formats, and regional compliance variations. To affect a sampling strategy based solely on english-based text would limit the program’s ability to drive value across every customer engagement.
Conceptualizing and deploying an IDP program that delivers consistently accurate results across various document formats doesn’t have to be an uphill struggle. However, enterprise leaders must stop viewing IDP as a simple point solution and acknowledge that IDP has many moving parts, all of which need to be orchestrated across the value chain.
Using AI tools to find patterns in large document databases quickly keeps your document pipeline running smoothly. Contract review affords an excellent example of how AI can be used to make document discovery easier. For example, training an AI solution to tag different clauses within specific contract sets makes it much easier for contract review specialists to flag high-risk documents for review at scale.
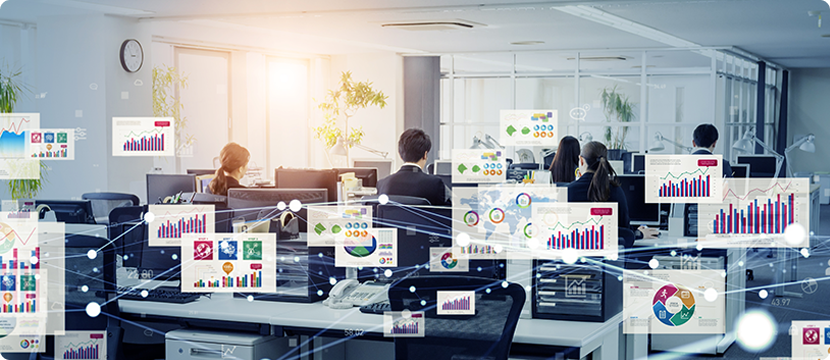
Building a custom AI model isn’t just time-consuming and can make model-building harder down the line when document formats change. However, pre-trained models have a running start when understanding data markers and can be optimized much faster. They usually require much fewer data than a custom build to get started on document workloads.
Working with a leading US bank to automate their KYC document processing, we pre-trained a model in our environment while installing an IDP application on their on-premise hardware. Once the application was up and running, we lifted and shifted the pre-trained models to the bank’s infrastructure and put it to work. The result was that they began to see returns in just three months instead of the year-long training time that many complex, custom-built AI models typically incur.
A unified framework makes automated testing easier, improves development traceability, and makes for shorter release cycles. A unified framework is almost essential to ensuring every cog turns in unison for complex IDP deployments that use a mélange of technologies to identify page layouts, character variations, and non-standard language use.
A faster route to IDP deployment involves partnering with your BPM providers to create a domain-based sampling strategy for AI models. For example, when we worked with a major US telecom provider to automate their contract review process for tower leases, their BPM vendor provided invaluable assistance in labelling data and layout variations and validating our model predictions. By harnessing their domain expertise and our solution capabilities, the firm’s contract review teams improved productivity by a whopping 60% while raking in $21 million in savings.
Loved what you read?
Get practical thought leadership articles on AI and Automation delivered to your inbox
Loved what you read?
Get practical thought leadership articles on AI and Automation delivered to your inbox
Successful IDP projects can improve decision speed and data visibility for business units, teams, and individuals alike. But enterprises must create a strong alignment between IDP project goals and desired business outcomes to get there. The trick is to focus on end-users – what are their needs from documentation, and how can an IDP platform deliver on those needs accurately and seamlessly? Organizations that successfully answer that question will have taken the first step toward IDP, continuously driving better outcomes instead of turning into a low-value cost centre.
Disclaimer Any opinions, findings, and conclusions or recommendations expressed in this material are those of the author(s) and do not necessarily reflect the views of the respective institutions or funding agencies