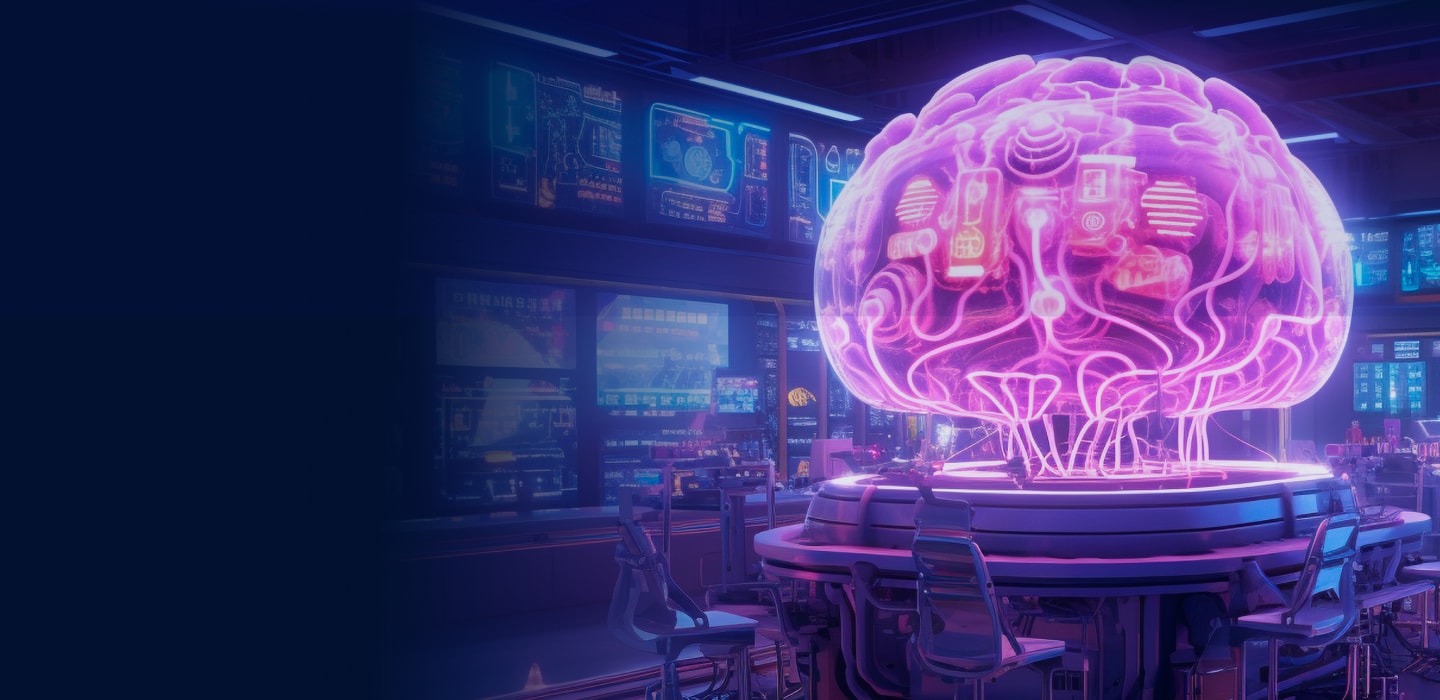
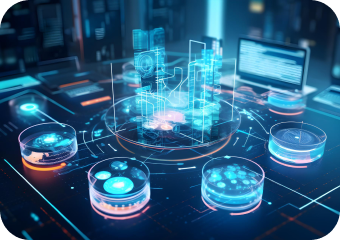
The enthusiasm for AI has led to fragmented efforts1, escalating costs, and missed opportunities. To unlock AI’s full potential, enterprises must adopt a centralized, platform-based approach that enables scalable, sustainable, and impactful AI deployment.
AI adoption has often taken a federated approach, where different departments or teams pursue isolated initiatives. Imagine an organization where five different departments are independently exploring AI-driven solutions for customer service, logistics, marketing, HR, and IT. Each team uses different tools, contracts with different vendors, and develops separate models.
While this decentralized experimentation allows for rapid innovation, it brings with it significant challenges: Duplication of efforts across teams. Cost overruns due to a lack of visibility into resource usage and financial implications. Variability in quality and performance due to disparate tools and methodologies. Weak data governance and compliance controls.
The Centralized Alternative
Contrast this with a centralized AI platform. Departments share a common infrastructure, tools, and governance framework. Instead of starting from scratch, teams can leverage pre-built models, shared data repositories, and standardized APIs to accelerate development. A platform approach2 directly address the shortcomings of a federated approach:
-
Unified Governance
Centralization ensures that data sources, model usage, and compliance frameworks are uniformly managed. This minimizes risks associated with legal, ethical, and regulatory requirements while maintaining a high standard of quality across all AI initiatives.
-
Cost Efficiency
By consolidating resources, enterprises can eliminate redundant expenditures on infrastructure, tools, and talent. A shared platform allows for optimized resource allocation, ensuring that every dollar spent contributes to measurable outcomes.
-
Scalability and Agility
A unified platform approach as opposed to a web of point solutions abstracts complexity, enabling teams to focus on outcomes rather than underlying technologies. With standardized APIs and reusable components, enterprises can rapidly deploy AI solutions across diverse use cases.
-
Flexibility for Future Innovations
AI technology evolves rapidly. Centralized systems provide the modularity needed to integrate emerging models, tools, and infrastructure without disrupting existing workflows.
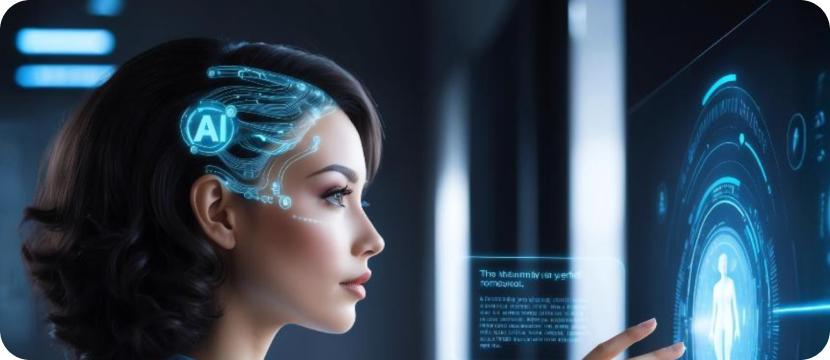
Building Blocks of Intelligent Platforms
Platforms3 have the power to turn big ideas into reality. But scaling any technology comes with its fair share of pitfalls. Organizations often find themselves pouring resources without mapping a clear pathway to production, resulting in wasted effort and diminishing returns. To avoid this misstep, platforms should be designed with modularity4 in mind. The focus should remain squarely on delivering tangible business value, whether that means enhancing operational efficiency, improving customer experiences, or unlocking new revenue streams. To realize the benefits of centralization, enterprises must build a robust platform that integrates key elements:
-
Infrastructure Layer: The Muscle Behind the Machine
Infrastructure is where the heavy lifting happens5. It’s the layer that crunches numbers, stores mountains of data, and keeps everything running without falling apart. But here’s the catch: it’s not enough to just have powerful servers or a shiny cloud subscription.
A real intelligent platform knows how to handle everything from massive model training jobs to lightweight edge computing. Whether it needs to analyze real-time sensor data or process a billion records, the infrastructure needs to scale up or down without making a fuss. All this should happen without anyone even noticing—dynamic provisioning ensures developers never have to think about where resources are coming from. The infrastructure just gets it done.
-
Model Management Layer: The Brainpower
The model management layer is where the AI brains live. This is more than just a storage room for models; it’s a carefully curated brain trust. Imagine having hundreds of models across different teams. Without a system to manage them, we would end up retraining the same model multiple times or worse, deploying an outdated one that makes decisions based on two-year-old data. The model management layer eliminates this. It tracks versions, monitors performance, and flags what’s working (and what isn’t).
But the real magic lies in continuous learning. Models don’t just sit there collecting digital dust—they evolve. The platform creates feedback loops so models improve over time, adapting to new data and environments.
-
Data Layer: The Lifeline of AI
AI without good data is like a race car without fuel. The data layer ensures the platform gets exactly what it needs: clean, labeled, structured data delivered on time.
But, enterprise data is messy. It’s scattered across silos, stored in multiple different formats, and imprisoned. The data layer solves this by centralizing access while keeping governance tight. It makes sure the right people and models get the right data while keeping everyone else out.
-
API Abstraction Layer: The Great Simplifier
AI doesn’t need to be complicated. The API abstraction layer6 makes sure it isn’t. This is the layer that hides all the messy details—complex algorithms, vast datasets—and serves up simple, user-friendly APIs.
Want personalized product recommendations? Just call an API. Need sentiment analysis? There’s an API for that too. The brilliance of this layer is that it turns sophisticated AI capabilities into plug-and-play features anyone can use.
And it’s not just about simplicity. These APIs are reusable and scalable, meaning the marketing team can roll out a personalization engine while the HR team uses the same platform for employee sentiment analysis. No reinventing the wheel, no wasted time.
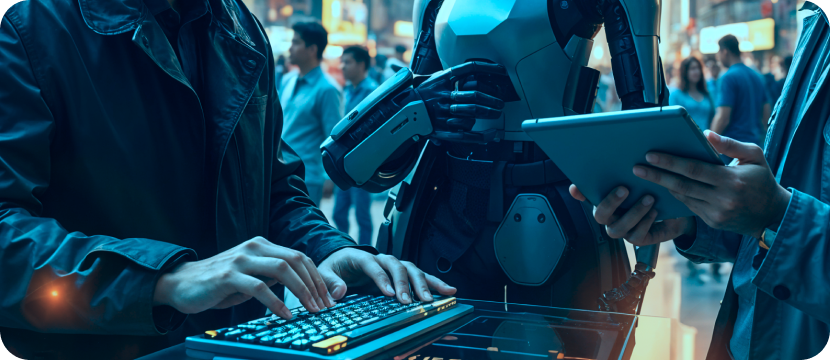
-
Governance Layer: The AI Traffic Cop
The governance layer7 is the traffic cop of the platform, ensuring everything runs smoothly and stays within the lines. This is where compliance lives. Whether it’s GDPR, HIPAA, or internal ethical guidelines, the governance layer enforces the rules. It makes sure the models are traceable, the data is secure, and the decisions are explainable. If an AI model recommends rejecting a loan, the governance layer can show exactly why that decision was made.
It’s not just about avoiding lawsuits; it’s about building trust. With governance baked in, the teams and stakeholders can rely on AI decisions without second-guessing their fairness or legality.
-
User Experience Layer: The Face of the Platform
The experience layer is the part of the platform users actually see. This is where design meets intelligence. Whether it’s a drag-and-drop workflow builder, a real-time performance tracker, or a chatbot interface, the experience layer makes AI approachable. It ensures that everyone—not just data scientists—can leverage the platform to solve problems and drive impact.
This layer is the bridge between technology and people, turning abstract AI capabilities into practical solutions anyone can use.
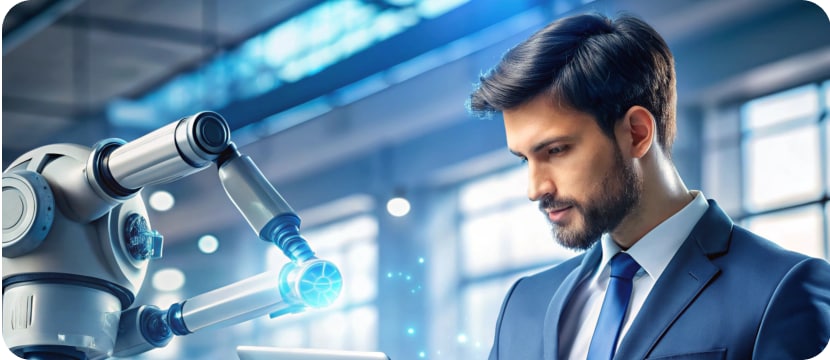
Loved what you read?
Get practical thought leadership articles on AI and Automation delivered to your inbox
Loved what you read?
Get practical thought leadership articles on AI and Automation delivered to your inbox
Scalable Collaborative AI: Build Once, Scale Forever
Scaling AI requires more than ambition—it demands structure, governance, and strategic foresight. A centralized platform approach provides the foundation for enterprises to:
- Govern AI effectively across diverse use cases.
- Manage costs and ensure sustainable ROI.
- Adapt to rapid technological advancements with ease.
Enterprises that embrace this approach will be anti-fragile against any technological advancements or disruptions. The time to act is now—before the inefficiencies of fragmented experimentation become insurmountable.
Disclaimer Any opinions, findings, and conclusions or recommendations expressed in this material are those of the author(s) and do not necessarily reflect the views of the respective institutions or funding agencies.
- https://www.bcg.com/publications/2024/what-gen-ais-top-performers-do-differently
- https://www.mckinsey.com/capabilities/mckinsey-digital/our-insights/investing-in-the-future-of-tech-lessons-from-winning-companies
- https://www.forbes.com/councils/forbesbusinesscouncil/2024/07/08/how-to-fix-your-digital-transformation/
- https://www.mckinsey.com/capabilities/mckinsey-digital/our-insights/a-data-leaders-operating-guide-to-scaling-gen-ai
- https://www.mckinsey.com/industries/technology-media-and-telecommunications/our-insights/ai-power-expanding-data-center-capacity-to-meet-growing-demand
- https://www.mckinsey.com/capabilities/mckinsey-digital/our-insights/the-big-product-and-platform-shift-five-actions-to-get-the-transformation-right
- https://www.mckinsey.com/capabilities/quantumblack/our-insights/building-ai-trust-the-key-role-of-explainability