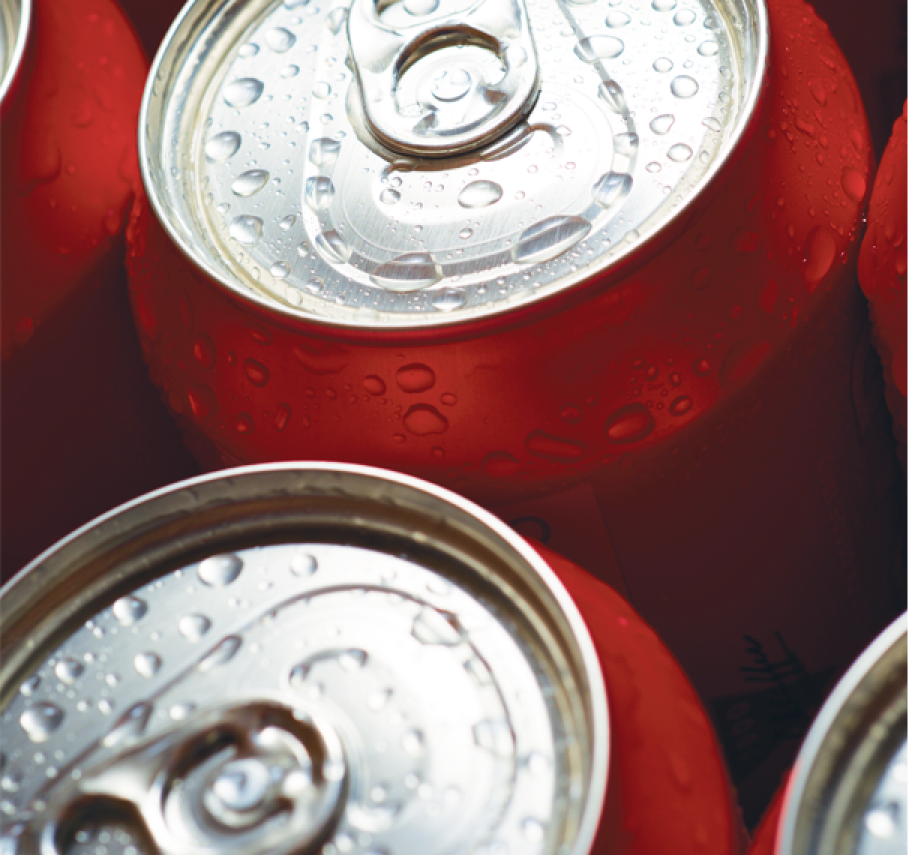
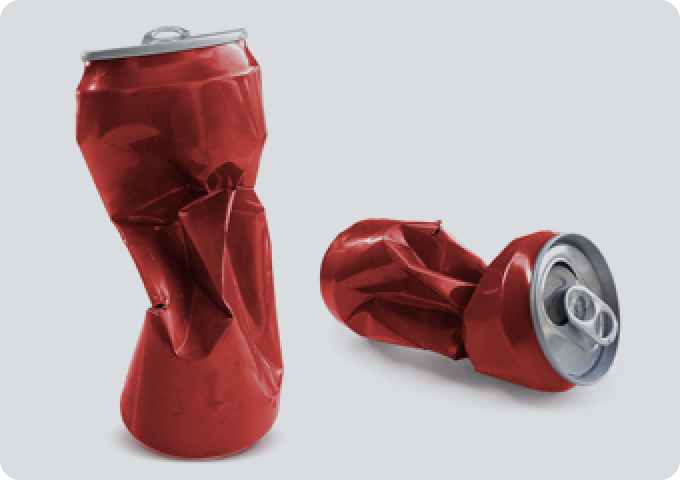
For most business leaders, the why of automation is pretty straightforward — cost savings, operational efficiencies, error-free processing, etc., The biggest challenge, however, is in what and how. The answer to these questions begins with robust process discovery. Read on to learn how you can strengthen your robotic process automation initiatives.
At its foundation, robotic process automation (RPA) automates an existing process step by step. So, any RPA initiative is only as good as the process that it automates. No business process is perfect, so this opens up a can of worms. Even everyday tasks like invoice processing, billing, cash management, etc., can be inconsistent from one to the next and need considered interventions.
In the manual world, people use their domain expertise and business intelligence to make adjustments for anomalies in the process. Except, this creates myriad, minor yet significant variations to existing processes. Such processes can be difficult to automate for several reasons:
- Variations often remain tacit knowledge, documented only in the minds of employees, and almost impossible to track
- Most variations are only needed sporadically and used by a specific few, making them easy to miss during process discovery
- Some variations might apply only to specific business units or geographies; therefore, they are unsuitable for global automation
While RPA has been successful with rule-based, high-volume, repetitive tasks, its impact has been limited when the processes are non-standardized and require frequent human intervention. The consequences of automating sub-optimal processes can be significant: Ranging from teams continuing to perform most of the tasks manually to abandoning the automation initiative altogether.
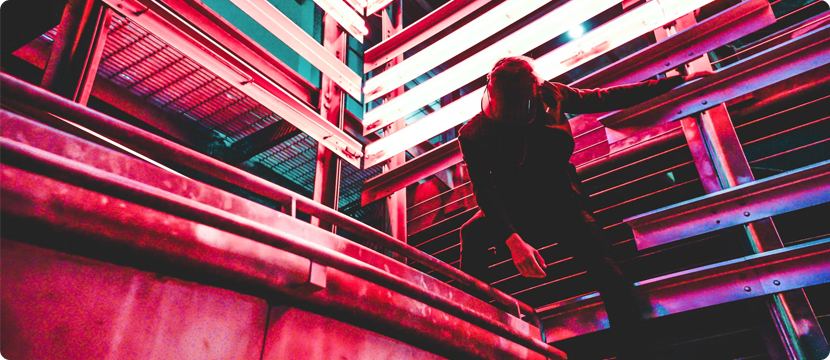
Process discovery forms the very foundation of effective robotic process automation. Good process discovery needs to collect process and task-level data from user devices, analyze it, provide insights and create a blueprint for automating the right processes.
Performing this manually is often an impossible task. Typically, analysts or consultants begin by interviewing teams and capturing the process flow. This is both time-consuming and expensive.
Often, there is resistance among team members to share information, which can be detrimental to holistic process discovery. Moreover, even when they are willing to share information, edge cases are often missed because users either don’t remember or the sample group doesn’t know.
On the other hand, manual process mapping misses data about crucial metrics such as process performance, stability, business exceptions, failure statistics, etc. Without these, there is no productive way to prioritize processes for automation.
Effective process discovery depends almost entirely on covering all the bases discussed above. It must be automated, cost-effective, comprehensive, and data-driven. Most importantly, it must also be accelerated to realize business outcomes.
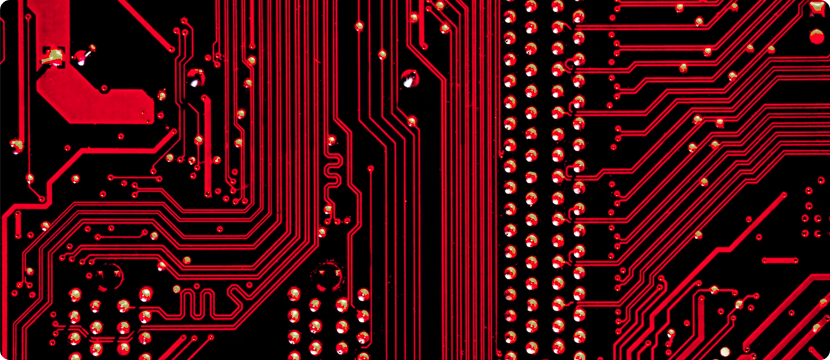
In our experience working with some of the largest enterprises across the globe, the necessary features of effective process discovery are:
- Intelligent data capture of granular process nuances using computer vision and keystroke logs
- Adaptable data capture with high configurability, ability to run offline, optimized data volume transmissions, and security features
- Process recording so that users can record the application usage and business flow for any process with start and end markers
- Legacy system support to ensure all processes and aspects are considered
- Interactive process visualization with process maps to trace as-is processes in terms of design, variations, and patterns
- Automation blueprints developed on the business insights obtained from the task and process-level data
- Automation prioritization using indicators, priority matrix, and smart funnel
In reality, this is how it works. The discovery tool captures users’ keystroke data by running on desktops and laptops in the background. Without disrupting work, these bots collect data on how the organization uses various applications and their interactions. Based on this data, the tool suggests the best processes for automation and creates corresponding workflows.
A leading global beverage company with operations across 20+ countries was looking to optimize and automate its cash collection and route settlement & billing processes. Due to their vast global operations, they faced several complexities with differences in local procedures, languages, and legal requirements. Process variations created non-standardization, limiting visibility and significantly increasing the cost of operations.
The client wanted to assess the various versions of processes, identify the optimal process, and standardize, centralize, and automate to lower costs and improve efficiencies.
Loved what you read?
Get practical thought leadership articles on AI and Automation delivered to your inbox
Loved what you read?
Get practical thought leadership articles on AI and Automation delivered to your inbox
We used this data to identify over 300 process variations, evaluate the best approaches, and spot the right opportunities for automation. Based on this insight, we designed an optimal process flow that would significantly improve the current process and decrease the cost of operations.
Outcomes
74
Processes
identified for
improvement
16
Processes
identified for
automation
$1M
Potential
savings
annually
65,000
Estimated reduction
in person-hours
annually
Deloitte found that RPA delivers a return on investment within 12 months. 92% of respondents reported improved compliance, 90% reported improved quality/accuracy, 86% reported improved productivity, and 59% said they realized cost reduction.
The primary reason for failed RPA implementations tends to be picking the wrong processes, not understanding the entire scope of the process, or not automating them comprehensively. All these challenges can be overcome with robust process discovery.
Whether you’re embracing RPA afresh or looking to optimize existing automation, begin your RPA journey with cost-effective, comprehensive, data-driven, accelerated, and AI-powered process discovery.
Disclaimer Any opinions, findings, and conclusions or recommendations expressed in this material are those of the author(s) and do not necessarily reflect the views of the respective institutions or funding agencies