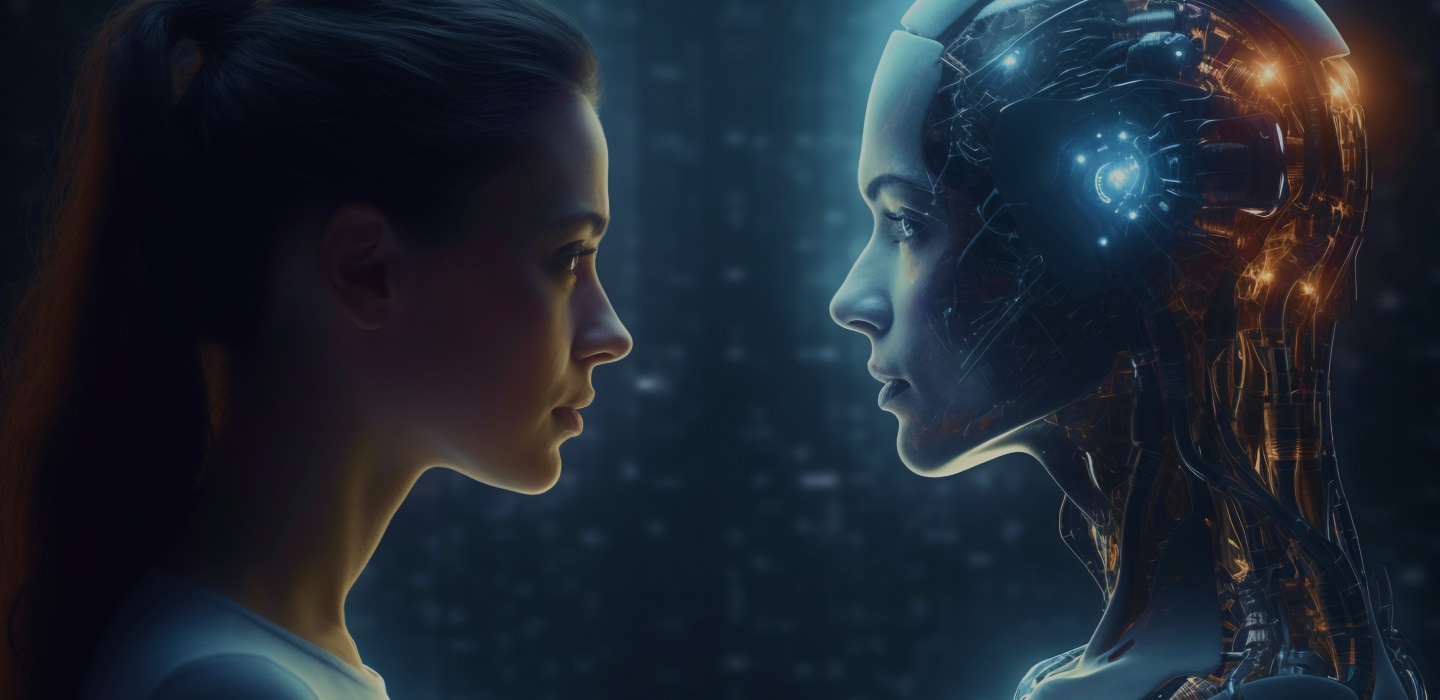
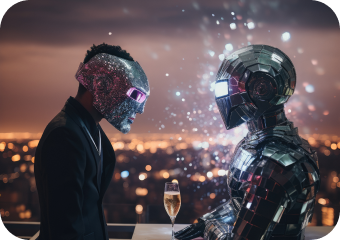
Generative AI (GenAI) is commanding the corporate spotlight, not just because it’s another shiny tech trend, but because it flips the traditional narrative of technology adoption on its head. This isn’t about IT teams or specialists—it’s about turning everyday professionals into creators. GenAI makes technology adoption so easy that everybody can actually do it.
This democratization has opened floodgates for enterprise use cases that once languished in the shadows. Ideas shelved due to complexity or cost are now being dusted off and given a second life, empowered by the accessibility and versatility of GenAI. Adoption rates have surged, with AI usage climbing from 50% in 2023 to a staggering 72%1 in 2024. Over 63%2 of executives now rank GenAI implementation as a “high” or “very high” priority.
But here’s the inconvenient truth: priority doesn’t equal payoff.
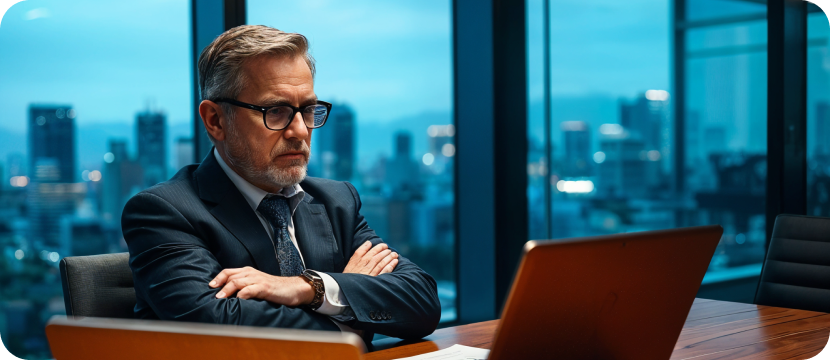
For every glowing headline about AI transformation, there’s a sobering statistic lurking in the background. Gartner’s forecast is a reality check: 30% of GenAI experiments will be abandoned after proof of concept. And that’s not a surprise when you consider this—70%3 of digital transformation initiatives fail outright. Even among those that survive, only 26%4 deliver tangible value.
This is where the rubber meets the road for business leaders. Yes, GenAI promises to empower creators, streamline operations, and revolutionize customer experiences. But promises don’t pay bills. For CFOs, every dollar spent must justify itself, not in theoretical potential but in concrete ROI.
As McKinsey puts it ‘the honeymoon phase of experimentation is over5.’ GenAI must now prove itself in the real world, where the business case reigns supreme. Enterprises aren’t chasing the hype anymore—they are chasing results, especially in the form of Operational Excellence (OPEX).
What should leaders do to ensure this operational excellence and will GenAI deliver enough value to justify the investment?
Operational excellence today is not just about efficiency—it’s about resilience and agility in an environment where change is the only constant. And to achieve that, leaders must address the foundational elements of OPEX: people, technology, data, and processes6.
“Whatever you don’t transform becomes the weakest link, and that will determine how well your operating model performs.”
– N Shashidhar,
Vice President and Global Platform Head at EdgeVerve
People: From Shadow IT to Empowered Innovators
For decades, businesses struggled with “architectural inertia”—a result of sprawling shadow IT systems created by employees bypassing slow-moving traditional IT structures. While this quick-fix approach enabled some agility in the short term, it ultimately crippled organizations’ ability to pivot quickly. Long tail use cases, fragmented systems, and patchwork solutions became roadblocks to innovation.
The modern OPEX model demands a fundamental shift: empower employees with technology rather than letting them circumvent it. GenAI is a catalyst here, making complex tech accessible and actionable for non-technical users. But the technology alone isn’t enough—it’s about collaboration.
A human-in-the-loop approach allows experts to validate AI outputs, injecting context and domain knowledge where algorithms fall short. Equally important is involving employees early in the GenAI rollout. Excluding the workforce risks leaving them unprepared for the shifts AI brings—not just operationally, but strategically and ethically. Employees need to understand how these tools impact their roles, contribute to decisions, and navigate complex ethical considerations.
Democratize technology, embed human expertise where it matters, and ensure your workforce is ready to co-create the future of your operating model. Otherwise, agility remains an elusive dream.
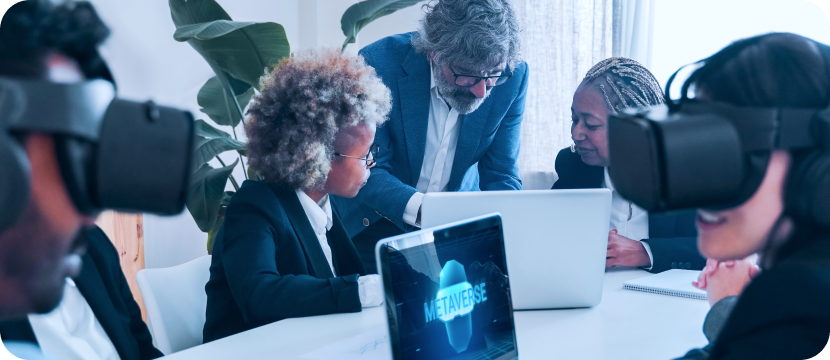
Technology: Architecture for Perpetual Innovation
GenAI has obliterated the boundaries of what technology can achieve, resurrecting use cases once discarded as impractical. But to capitalize on this, enterprises must think beyond mere upgrades—they must reimagine their technology stack.
This is where AI-first architecture comes into play. By migrating to scalable, cloud-based platforms that support API-driven access to GenAI models, companies are building a technology foundation designed for perpetual evolution.
But, generic tools no longer cut it. Closed, pre-trained models often fall flat against nuanced, domain-specific challenges. The real value lies in fine-tuning foundational models7 using industry-specific data and context. This tailored approach transforms technology from a tool into a competitive advantage.
True business value lies in looking beyond the narrow lens of individual use cases. Instead, enterprises must take an end-to-end approach, using GenAI as an enabler to reimagine workflows, automate redundancies, and optimize value chains. This is how you unlock real value from AI and create business cases for continued investment.
Enterprises that succeed in this transformation don’t rely on GenAI in isolation. They adopt a platform-based approach that integrates AI seamlessly with complementary technologies, such as RPA, Low-code development, workflow orchestration and document AI.
Data: Aligning Insights with the Speed of Decision Making
If technology is the engine, data is the fuel—but not just any fuel. In the post-big-data era, the challenge isn’t volume; it’s usability. Enterprises are sitting on mountains of data, much of it unstructured and untapped. GenAI shifts the narrative, turning data into a strategic asset—but only if it’s curated and activated effectively.
What was previously sparse was the ability to access, search, and reason over data, particularly unstructured data.That gap is closing. Emerging tools now make it possible to extract actionable insights from vast, disparate data sources.
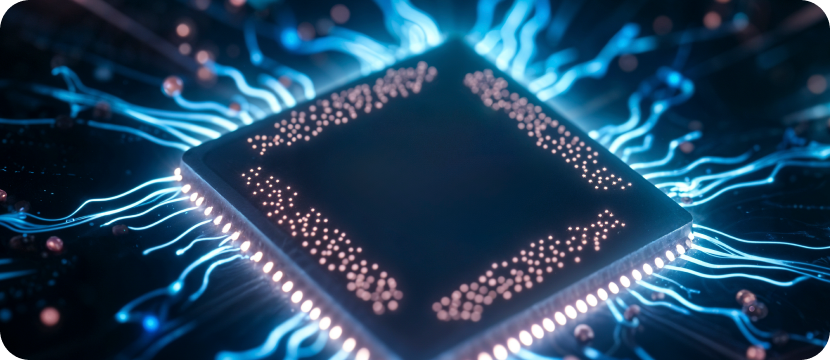
But the real breakthrough lies in data governance and agility. GenAI thrives on quality and timeliness, which means companies must establish resilient, real-time data pipelines. Data-as-a-service frameworks help ensure data flows seamlessly across systems, teams, and applications.
Stop treating data as an operational byproduct. Instead, view it as a high-octane resource that powers innovation. Establish governance, prioritize real-time flows, and invest in contextual relevance. This is how data shifts from being overwhelming to being indispensable.
Process: Eliminating Inner Efficiencies
Technology without process transformation is like adding horsepower to a car with a flat tire—it gets you nowhere fast. GenAI holds immense potential, but without addressing process debt, that potential is squandered. If we want to automate but don’t change the ways we are working, we are only accelerating the inner inefficiencies.
Point solutions, the most common entry point for enterprises experimenting with AI, rarely deliver sustainable ROI. These fragmented approaches are expensive, limited in scope, and often lead to stalled initiatives. The solution is using GenAI to reimagine and rebuild entire processes and value chains.
This integrated approach delivers more than just speed—it creates a smaller infrastructure footprint, centralizes governance, and establishes guardrails to ensure ethical and effective AI deployment.
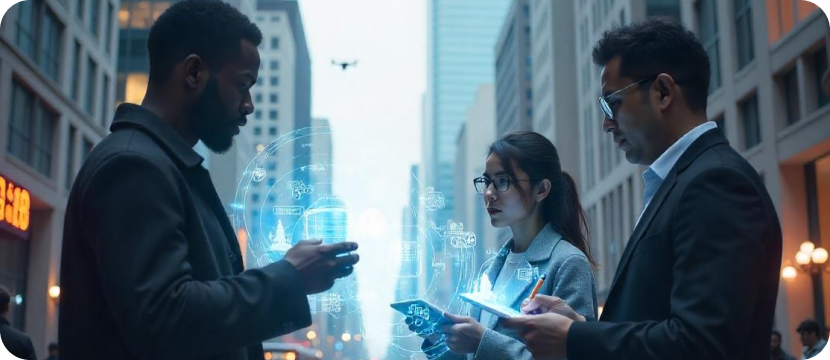
The benefits of a operational excellence mindset addressing all four pillars go beyond cost optimization or faster time-to-market. It establishes a scalable foundation for innovation, enabling enterprises to rapidly adapt as technology evolves. This agility is a critical differentiator in today’s competitive landscape.
Loved what you read?
Get practical thought leadership articles on AI and Automation delivered to your inbox
Loved what you read?
Get practical thought leadership articles on AI and Automation delivered to your inbox
For leaders looking to make a business case with operational excellence, here’s the three step golden formula.
- Address process debt before accelerating with AI.
- Shift focus from isolated point solutions to holistic transformation.
- Embrace platforms that integrate AI with other technologies for seamless scaling.
Disclaimer Any opinions, findings, and conclusions or recommendations expressed in this material are those of the author(s) and do not necessarily reflect the views of the respective institutions or funding agencies
- https://www.mckinsey.com/capabilities/quantumblack/our-insights/the-state-of-ai
- https://www.mckinsey.com/capabilities/risk-and-resilience/our-insights/implementing-generative-ai-with-speed-and-safety
- https://www.gartner.com/en/newsroom/press-releases/2024-07-29-gartner-predicts-30-percent-of-generative-ai-projects-will-be-abandoned-after-proof-of-concept-by-end-of-2025
- https://www.mckinsey.com/capabilities/mckinsey-digital/our-insights/tech-forward/why-most-digital-banking-transformations-fail-and-how-to-flip-the-odds
- https://www.bcg.com/press/24october2024-ai-adoption-in-2024-74-of-companies-struggle-to-achieve-and-scale-value
- https://www.mckinsey.com/capabilities/mckinsey-digital/our-insights/moving-past-gen-ais-honeymoon-phase-seven-hard-truths-for-cios-to-get-from-pilot-to-scale
- https://www.bcg.com/publications/2024/laying-tech-foundation-gen-ai-success