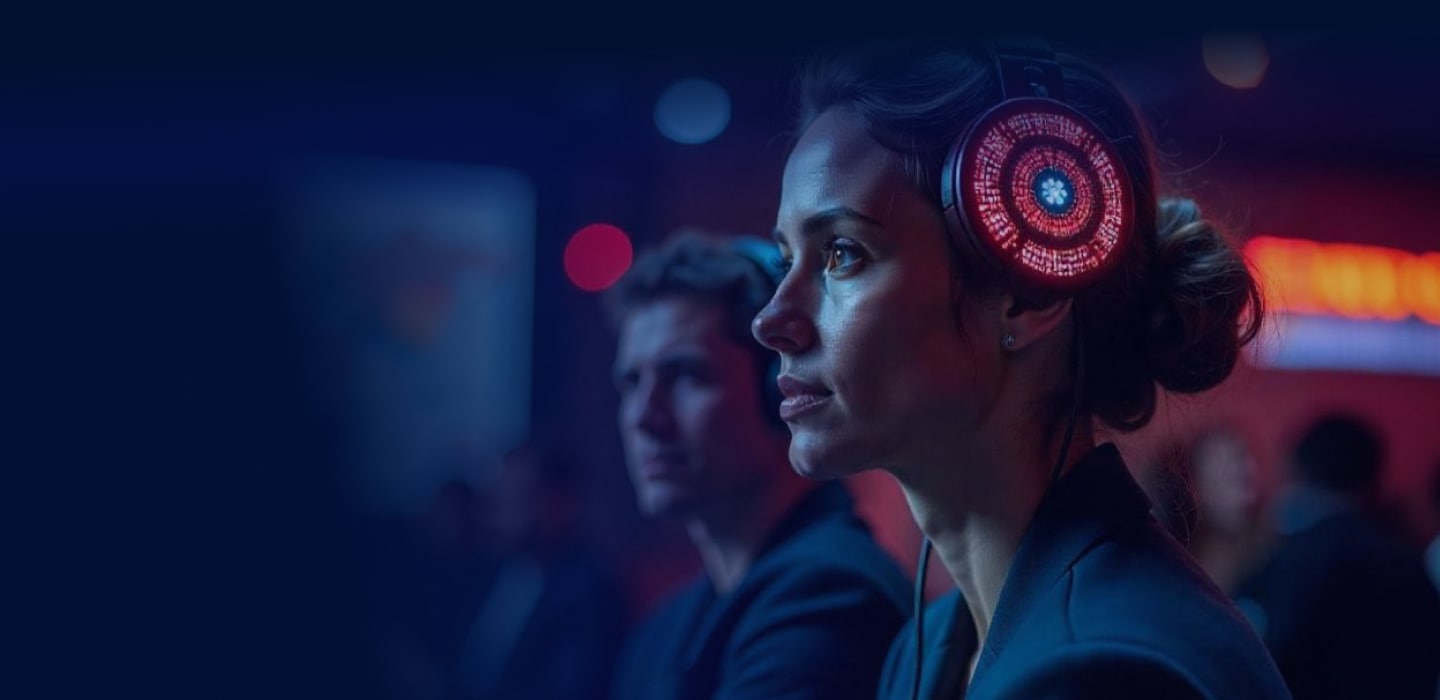
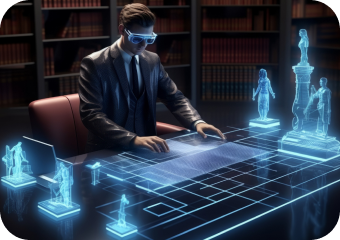
Technology no longer sits exclusively in the ivory towers of large enterprises. Today, it’s trickling down—empowering individuals, communities, and industries in ways we have only begun to grasp. The tools, frameworks, and platforms once reserved for the elite are now enabling innovation at every level of society. At the heart of this transformation lies a powerful concept: the agentic framework1.
Technology leaders like Microsoft and EdgeVerve, are building platforms that turn what was once the complex task of building AI-powered agents into something remarkably accessible. It’s not just about coding anymore; it’s about simulating, strategizing, and executing—almost as if entire companies can exist as virtual, self-governing ecosystems.
Sure, it’s an experimental model for now, but the implications for the real world are huge. Think of a farmer, with no prior technical expertise, tapping into the same agri-tech insights as multinational corporations. An AI agent could design an entire crop plan, optimize irrigation schedules, and forecast market trends.
But how do these AI agents actually work? What makes them capable of solving complex, real-world challenges while adapting to diverse industries and use cases?
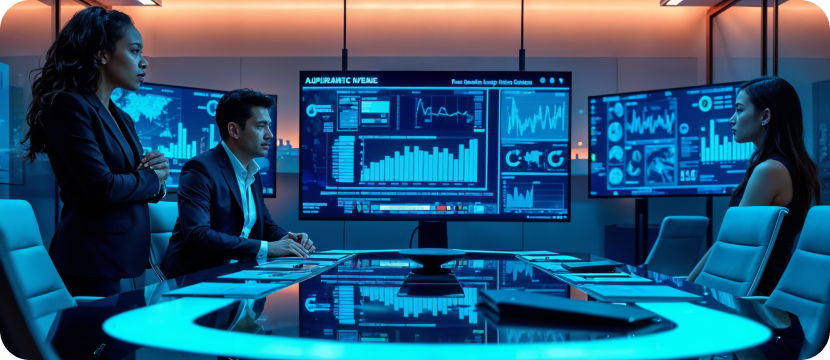
The Four Pillars of Agentic Frameworks: Data, Models, Agents, and Responsible AI
To truly understand and leverage agentic frameworks, we need to go deeper into the mechanisms that make them work. These systems rely on a symbiotic relationship between data, models, agents, and a robust governance layer to ensure responsible deployment. Let’s unpack this step by step.
- Data: The Foundation of Contextual IntelligenceEverything starts with data. It’s not just about volume—it’s about relevance. The data you have defines what your AI can achieve. For instance, if you are building a travel agent application, the quality of the experience hinges on a well-curated set of inputs: Geographical data, weather information, accommodation databases, local preferences, and cultural and logistical factors that shape a traveler’s choices.
The right data ecosystem transforms an AI agent from a basic task handler into a contextual2 problem-solver. The process demands access to diverse, interconnected datasets, ensuring every input enriches the agent’s understanding.
- Models: Choosing the Right Brains for the JobOnce the data is in place, the next critical step is selecting the model3 that aligns with your goals. This decision goes beyond picking a foundation model like GPT-4 or a Hugging Face deployment; it’s about understanding the specific capabilities each model offers.
- Domain-Specific Models: Tailored for industries like healthcare, agriculture, or logistics.
- Generalist Models: Capable of handling a broad variety of tasks but require fine-tuning for niche applications.
For instance, planning a travel itinerary might involve leveraging models that specialize in natural language understanding while layering them with APIs for data retrieval. Tools like Microsoft’s AI Foundry or GitHub’s model marketplace make this exploration seamless, allowing you to test and optimize models efficiently.
- Agents: The Orchestrators of ActionHere’s where the magic happens: intelligent agents bring your data and models to life. Agents act as the orchestrators4, executing tasks, managing workflows, and delivering outcomes. Think of them as microservices but elevated to a cognitive level.
For example, in a travel agent application, one agent queries geographical data for user-specified locations. Another agent interacts with hotel APIs to fetch real-time options. A third agent contextualizes these results based on traveler preferences and external factors like weather.
- Responsible AI: Guardrails for Safety and FairnessAI doesn’t operate in a vacuum; it interacts with people, processes, and systems. This makes governance and safety5 non-negotiable. Model evaluation helps with regular assessments to ensure accuracy, fairness, and robustness. Content moderation guards against harmful or inappropriate inputs and outputs. Ethical oversight ensures transparency, accountability, and adherence to industry standards.
With tech giants like Microsoft bringing ecosystems with advanced APIs and enterprise-ready assistant frameworks, businesses can take agentic innovation beyond experimentation to full-scale implementation.
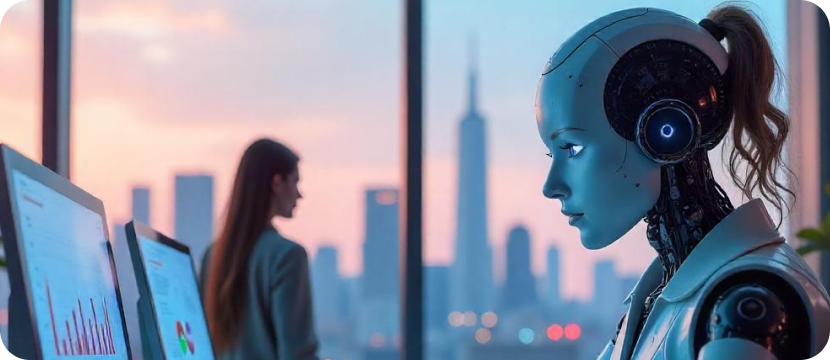
Will Agentic AI Replace Humans? A Reality Check
The question of whether agentic AI will replace humans isn’t new. The increasing autonomy of AI, combined with its ability to simulate decision-making and planning, makes the concern feel real. But let’s strip away the hype and look at the practical reality: AI operates in structured environments with well-defined rules. The real world doesn’t always work that way.
What’s fascinating is how AI systems are being implemented in stages, with clear milestones marking the evolution of their capabilities. This layered progression provides a roadmap for understanding AI’s role in augmenting human capabilities, not replacing them. Let’s explore this journey through five levels of AI implementation and what they mean for the future of work.
- Level 1: Chatbots and Task AutomationThe first wave of AI implementation was the chatbot. These systems streamlined simple, repetitive interactions—think customer service inquiries or basic transactional tasks. While limited, this marked the beginning of organizations experimenting with AI to free up human resources for more strategic work.
- Level 2: Enhanced Reasoning ModelsWe have already moved beyond basic chatbots into systems with advanced reasoning capabilities. With reinforcement learning and fine-tuned inference mechanisms, models like OpenAI’s GPT series have started handling complex planning tasks. For instance, these models can assist in crafting detailed project plans or conducting deep-dive analyses, but they still operate within predefined parameters.
- Level 3: Agents as OrchestratorsThis is where we are now. Agentic AI goes beyond executing commands; it orchestrates multiple interconnected tasks. For example, virtual assistants in IT operations can monitor systems, identify potential issues, and even implement fixes autonomously. Agents are becoming integral to industries where the tasks were traditionally managed by human operators—desk agents, schedulers, and administrative staff.
But even here, humans remain critical. Why? Because these agents thrive in structured, well-defined scenarios. When the unexpected arises, or when the context is deeply nuanced, human intervention becomes essential.
- Level 4: Specialized IntelligenceThe next step—often misinterpreted as the beginning of AGI (Artificial General Intelligence)—is specialized intelligence. AI models can already solve PhD-level problems in math or generate optimized code snippets for complex software development tasks. But does this mean they can replace humans in all domains? Far from it. These systems excel in narrow, domain-specific tasks. While they will outperform humans in specific scenarios, their limitations become evident when tasked with adapting to entirely new and undefined environments.
- Level 5: Automating Repetitive, High-Volume FunctionsAt the final level, we will see widespread automation of processes that are highly repetitive and rules-driven. Take resourcing in HR as an example:
- Scanning resumes.
- Filtering candidates based on job requirements.
- Scheduling interviews.
- Matching skills to roles using advanced reasoning models.
This is where AI’s potential for replacing humans peaks, but only in roles where creativity, empathy, and contextual understanding aren’t critical. Even here, humans will oversee, refine, and guide the AI systems to ensure alignment with organizational goals and values.
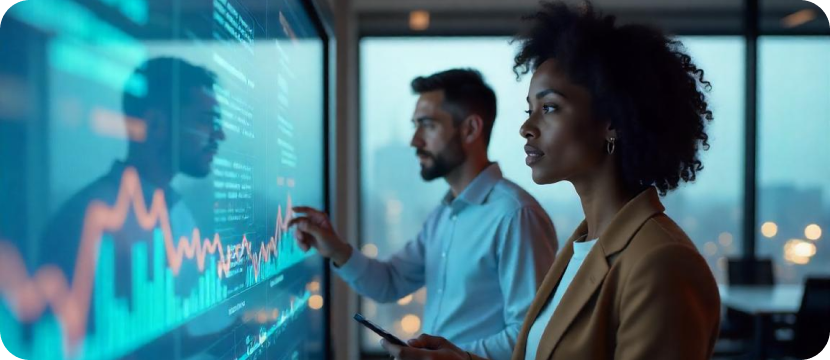
Loved what you read?
Get practical thought leadership articles on AI and Automation delivered to your inbox
Loved what you read?
Get practical thought leadership articles on AI and Automation delivered to your inbox
AI and Human Synergy: The Pragmatic Reality
The idea that AI will completely replace humans misses the bigger picture. Organizations don’t just need efficiency; they need empathy, creativity, and contextual intelligence—qualities that remain distinctly human. For example, industries with chronic staffing shortages—think healthcare or education—will rely on AI to fill gaps, allowing humans to focus on what truly matters. A hospital AI might handle scheduling and administrative workflows, freeing doctors to spend more time with patients.
The fast movers—those willing to experiment and scale—are creating ripple effects that extend far beyond their own walls. The tools are here, the frameworks are accessible, and the future is agentic.
Disclaimer Any opinions, findings, and conclusions or recommendations expressed in this material are those of the author(s) and do not necessarily reflect the views of the respective institutions or funding agencies.
- https://www.mckinsey.com/capabilities/mckinsey-digital/our-insights/why-agents-are-the-next-frontier-of-generative-ai
- https://www.mckinsey.com/industries/financial-services/our-insights/context-is-key-tanguy-catlin-on-advancing-the-future-of-insurance
- https://www.mckinsey.com/capabilities/mckinsey-digital/our-insights/what-every-ceo-should-know-about-generative-ai
- https://www.mckinsey.com/capabilities/mckinsey-digital/our-insights/why-agents-are-the-next-frontier-of-generative-ai
- https://www.mckinsey.com/capabilities/quantumblack/how-we-help-clients/generative-ai/responsible-ai-principles