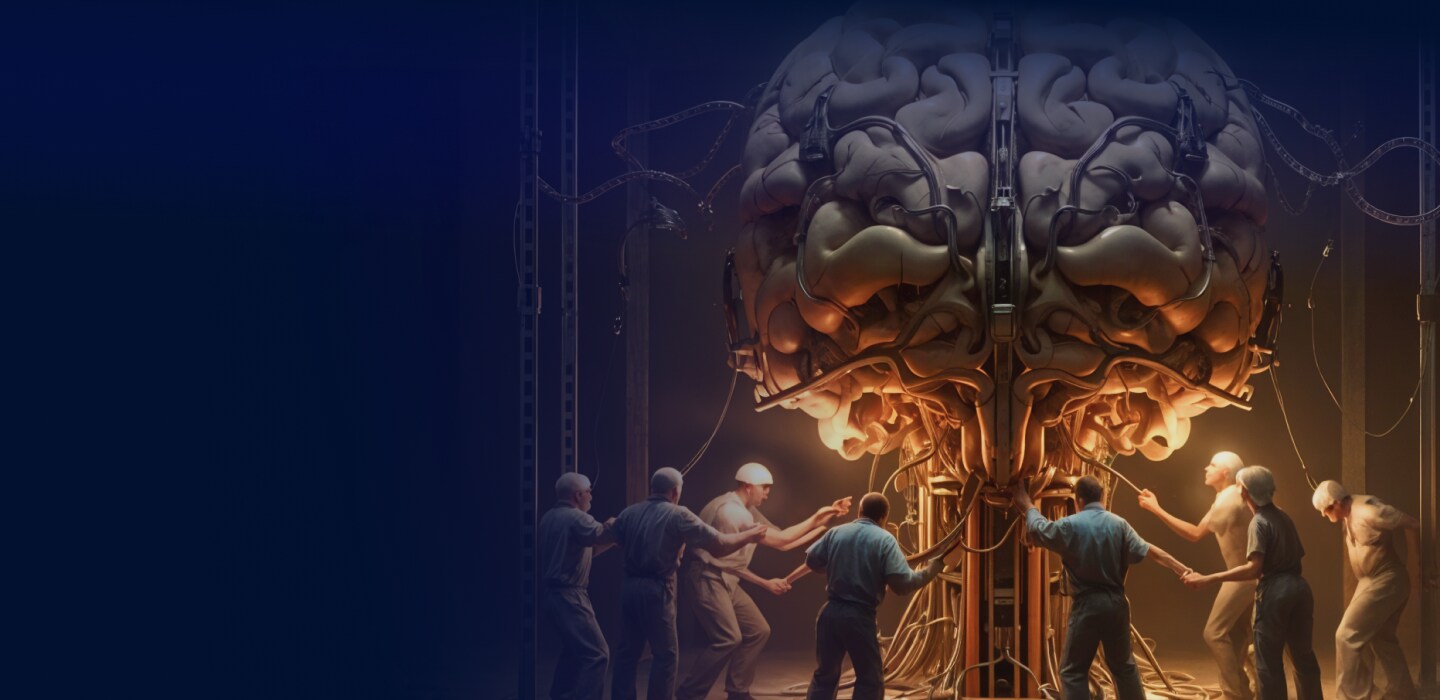
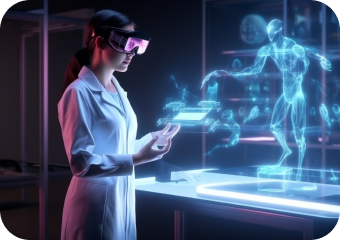
For decades, businesses have been drowning in data while starving for intelligence. Dashboards filled with numbers and graphs tell us what happened, but they rarely tell us why. The assumption has always been that if we collect enough data, patterns will emerge, and intelligence will follow. That assumption is flawed.
The real challenge isn’t data scarcity—it’s making sense of the chaos. True intelligence isn’t about collecting more numbers but about connecting the right dots. And so, the difference between leaders and laggards today isn’t access to data but their ability to extract meaning from it.
Data to Decisions: The Big Bottleneck
Every organization has structured data sitting neatly in rows and columns—sales figures, customer records, and inventory levels. Then there’s the unstructured data—the social media chatter, customer complaints, product reviews, economic trends, and competitor moves. Structured data tells you what’s happening inside your walls. Unstructured data tells you what’s coming next. Yet, most businesses remain trapped in the former1, running reports while the market moves on without them.
Predictive analytics attempts to solve this, but it operates within predefined parameters. It can forecast demand based on historical trends, but it struggles with the unexpected, like a competitor’s aggressive discounting, a sudden shift in consumer sentiment, or an economic downturn. That’s where the difference between intelligence and just data becomes apparent.
Take, for example, a retail chain tracking sales performance. Predictive AI can highlight a sudden surge in ice cream sales, but it takes an intelligent system incorporating external factors—weather forecasts, local events, and economic conditions—to recognize that an incoming heatwave is driving demand. Without this layer of intelligence, businesses are reactive, not proactive.
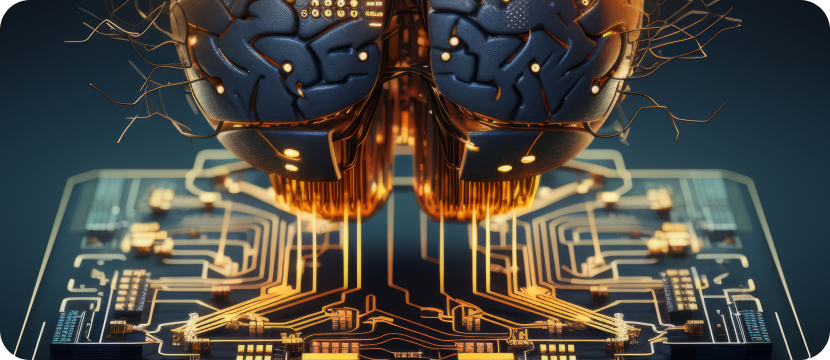
Predictive vs. Generative AI: The False Dichotomy
The conversation around AI often turns into a binary debate between predictive and generative models2. Predictive AI is structured, focused, and trained on historical data. It excels at spotting patterns, making forecasts, and improving efficiency. Gen AI, on the other hand, thrives in ambiguity. It synthesizes new ideas, interprets context, and uncovers insights buried in vast amounts of unstructured information.
Neither approach is sufficient on its own. Predictive AI can tell a retailer that ice cream sales spike in the summer, but generative AI can scan news reports and weather forecasts to explain why sales might deviate from expectations this year. One sees patterns; the other understands narratives. The real power lies in their fusion.
In banking, for example, predictive AI can assess a customer’s creditworthiness by analyzing their financial history, but generative AI can scan employment reports, inflation trends, and regional economic indicators to refine the risk assessment. By merging structured predictions with real-world context, institutions can move beyond one-size-fits-all risk models and craft more accurate financial products.
Intelligence Without Context is Useless
The conversation around AI often turns into a binary debate between predictive and generative models. Predictive AI is structured, focused, and trained on historical data. It excels at spotting patterns, making forecasts, and improving efficiency. Gen AI, on the other hand, thrives in ambiguity. It synthesizes new ideas, interprets context, and uncovers insights buried in vast amounts of unstructured information.
Consider supply chain management. A predictive model may highlight a spike in delivery delays. Useful? Yes. Actionable? Not without context. Generative AI can sift through port congestion reports, labor strikes, and geopolitical news to determine if the delays are systemic or temporary. Decisions must be made on intelligence, not just data points.
A gas utility company, for example, might use predictive AI to detect anomalies in meter readings, flagging a potential leak. However, without intelligence from external sources—temperature fluctuations, equipment wear-and-tear reports, and consumer behavior data—the system might generate too many false positives or miss emerging risks. Intelligence, not just analytics, is what separates a functioning system from a truly valuable one.
The Real Barrier is Data Readiness, Not AI Capability
AI is not the limiting factor. Poor data infrastructure is3. At least 70% of the enterprises have siloed data, incomplete records, and governance issues that make meaningful intelligence extraction nearly impossible. No AI model—predictive or generative—can fix bad data. Before investing in AI-driven intelligence, organizations must first:
- Break Down Data Silos – Ensure structured and unstructured data sources are integrated.
- Improve Data Quality – Inaccurate or incomplete data leads to flawed intelligence.
- Ensure Governance and Security – AI-driven decision-making requires clear rules on data access and usage.
Financial institutions, for example, often have fragmented records across various departments—mortgages, credit cards, retail banking. Without a unified view, they struggle to offer personalized financial services.
Organizations often approach AI expecting it to deliver revelations. It won’t. What it will do is amplify the quality of existing decision-making. If a company lacks strategic clarity, AI will only accelerate bad decisions. If the foundation is strong, AI will turn insights into competitive advantage.
Consider customer service. AI-powered chatbots and recommendation engines are only as good as the intelligence behind them. A bank using AI to analyze past interactions can anticipate customer needs—whether it’s detecting a frustrated caller based on previous service requests or proactively offering loan restructuring options based on economic forecasts. AI isn’t about automating decisions—it’s about enhancing human judgment.
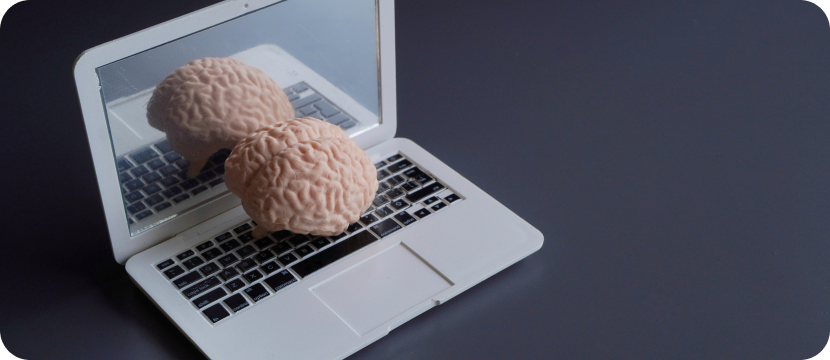
The Fusion of Data, Human and Machine Intelligence
Human intuition and AI-driven intelligence must work together. Sales managers, for instance, benefit from AI-driven insights, but their expertise in customer relationships, market intuition, and strategic thinking remains irreplaceable. A hybrid model—where AI handles pattern recognition while humans apply strategic judgment—ensures that businesses don’t blindly trust algorithms but use them as force multipliers.
This approach applies across industries. Healthcare professionals, for example, rely on AI for diagnostic support, but ultimate decision-making remains with the doctor. AI can analyze medical records and suggest treatment options based on similar cases, but human expertise ensures ethical considerations, patient preferences, and unforeseen complications are factored in.
Loved what you read?
Get practical thought leadership articles on AI and Automation delivered to your inbox
Loved what you read?
Get practical thought leadership articles on AI and Automation delivered to your inbox
Intelligence as an Unfair Advantage
The currency of power has shifted from capital and scale to insight and adaptability. The world’s most dominant businesses aren’t just data-rich; they are intelligence-rich, leveraging it to anticipate, adapt, and outmaneuver their competition4 before others even sense the shift.
The fundamental flaw in how most organizations approach AI is treating it as an efficiency tool rather than an intelligence amplifier. AI isn’t here to make businesses slightly faster or marginally better—it’s here to fundamentally change the way organizations perceive reality, enabling them to see beyond the obvious.
Disclaimer Any opinions, findings, and conclusions or recommendations expressed in this material are those of the author(s) and do not necessarily reflect the views of the respective institutions or funding agencies.
- https://www.mckinsey.com/capabilities/mckinsey-digital/our-insights/a-data-leaders-operating-guide-to-scaling-gen-ai
- https://www.ibm.com/think/topics/generative-ai-vs-predictive-ai-whats-the-difference
- https://www.mckinsey.com/capabilities/mckinsey-digital/our-insights/a-data-leaders-technical-guide-to-scaling-gen-ai
- https://www.technologyreview.com/2024/01/15/1086461/outperforming-competitors-as-a-data-driven-organization/